According to our upcoming study, 87% of marketers use AI for content. I’m one of them.
AI helped me build a career around writing. It’s part of nearly everything I do. Honestly, I can’t even remember how I worked without it—and I don’t want to.
It gives me a “second brain” and superhuman speed, and takes care of all the tedious tasks I dislike about my job.
Sure, maybe one day AI will replace my job. But right now? The real risk is not using it and falling behind everyone who is.
This guide shares everything I’ve learned from about two years of using AI in content marketing, often before my managers believed in it. I’ll show you when to lean into your human strengths and when to let AI take over.
AI is cheaper. About 4.7x cheaper than human-made content.
If you look at the distribution of content cost, you’ll see more AI users towards the left with the lower cost. Marketers who don’t use AI always pay more.
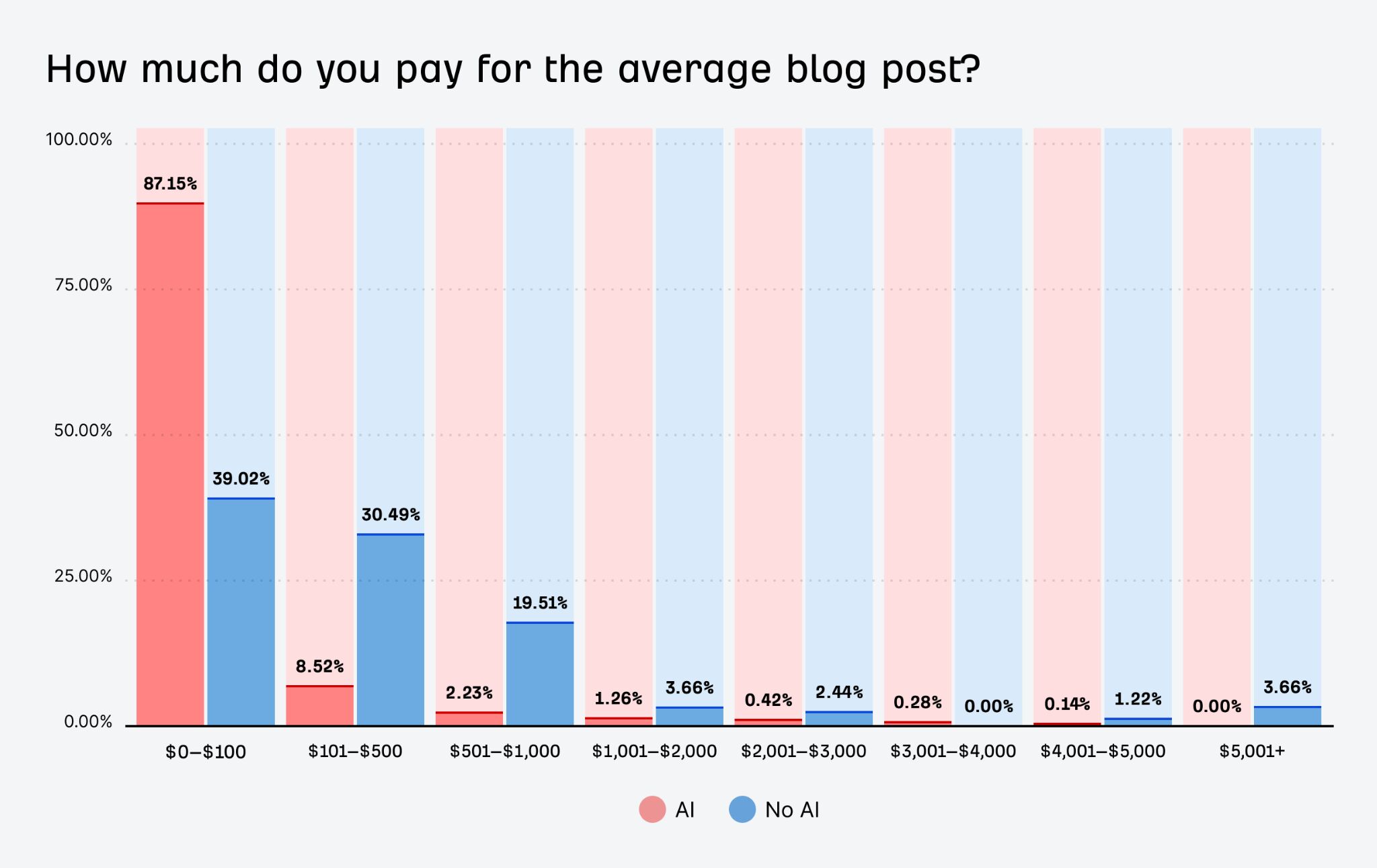
And AI makes everything cheaper, from blog posts to videos and whitepapers.
But is it actually any good, or are people just trying to cut down costs?
I’m going to give you first-hand examples of where AI is absolutely phenomenal, almost necessary. No theory, just tried and tested stuff I can’t imagine working without.
Come up with content ideas faster
AI can help you get past the blank page by suggesting topics, story angles, keywords for SEO, and headline variations. It’s a quick way to explore options and keep your content calendar full without scrambling for inspiration.
For example, in my guides, I always suggest starting keyword research by letting AI come up with the first ideas (aka seed keywords). Don’t worry if you’re not sure what those are—AI knows, and it’s surprisingly good at finding them. You can ask any large language model (LLM) to help, or just use the AI inside Ahrefs’ Keywords Explorer to instantly generate thousands of keyword ideas from those starting points.
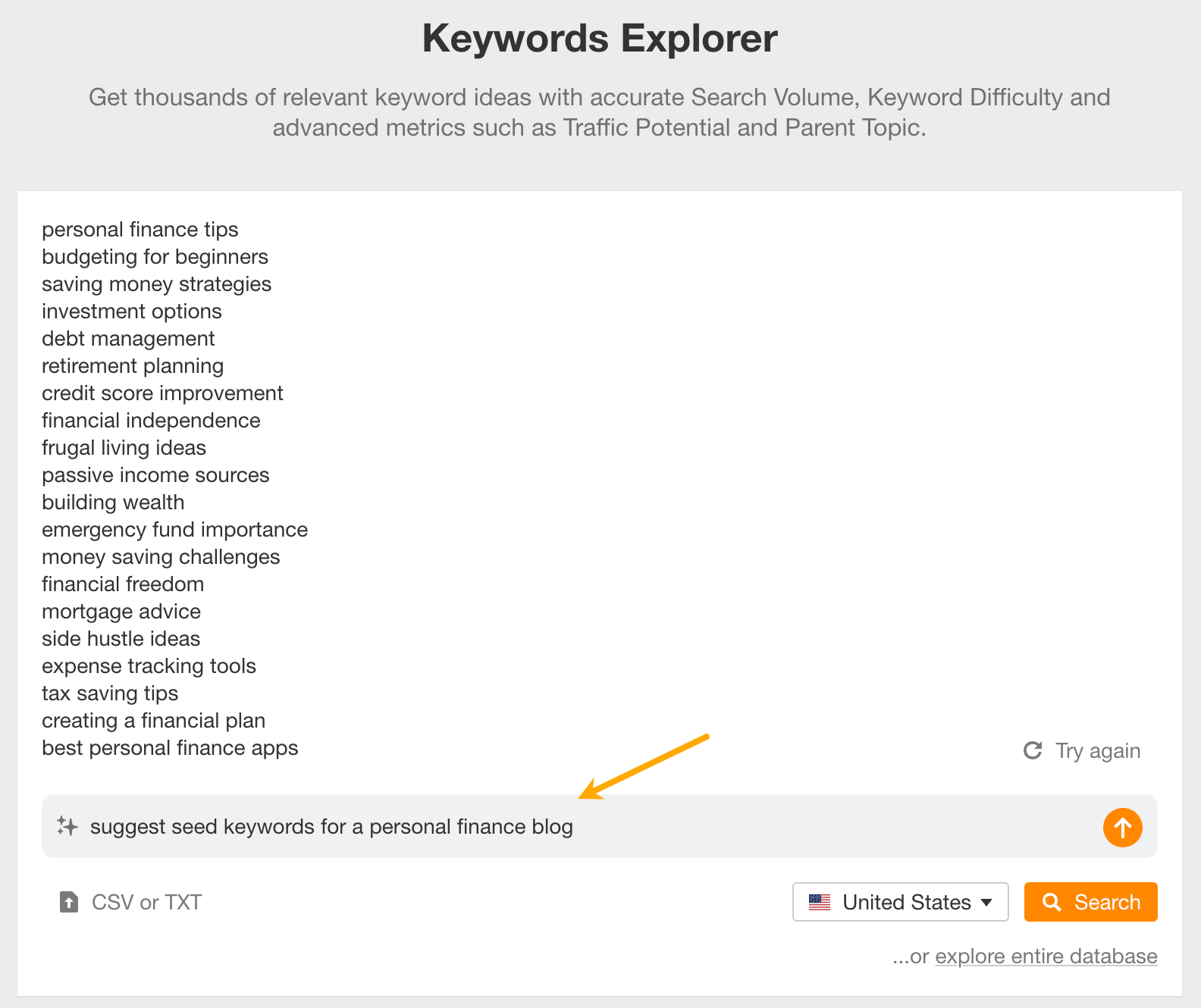
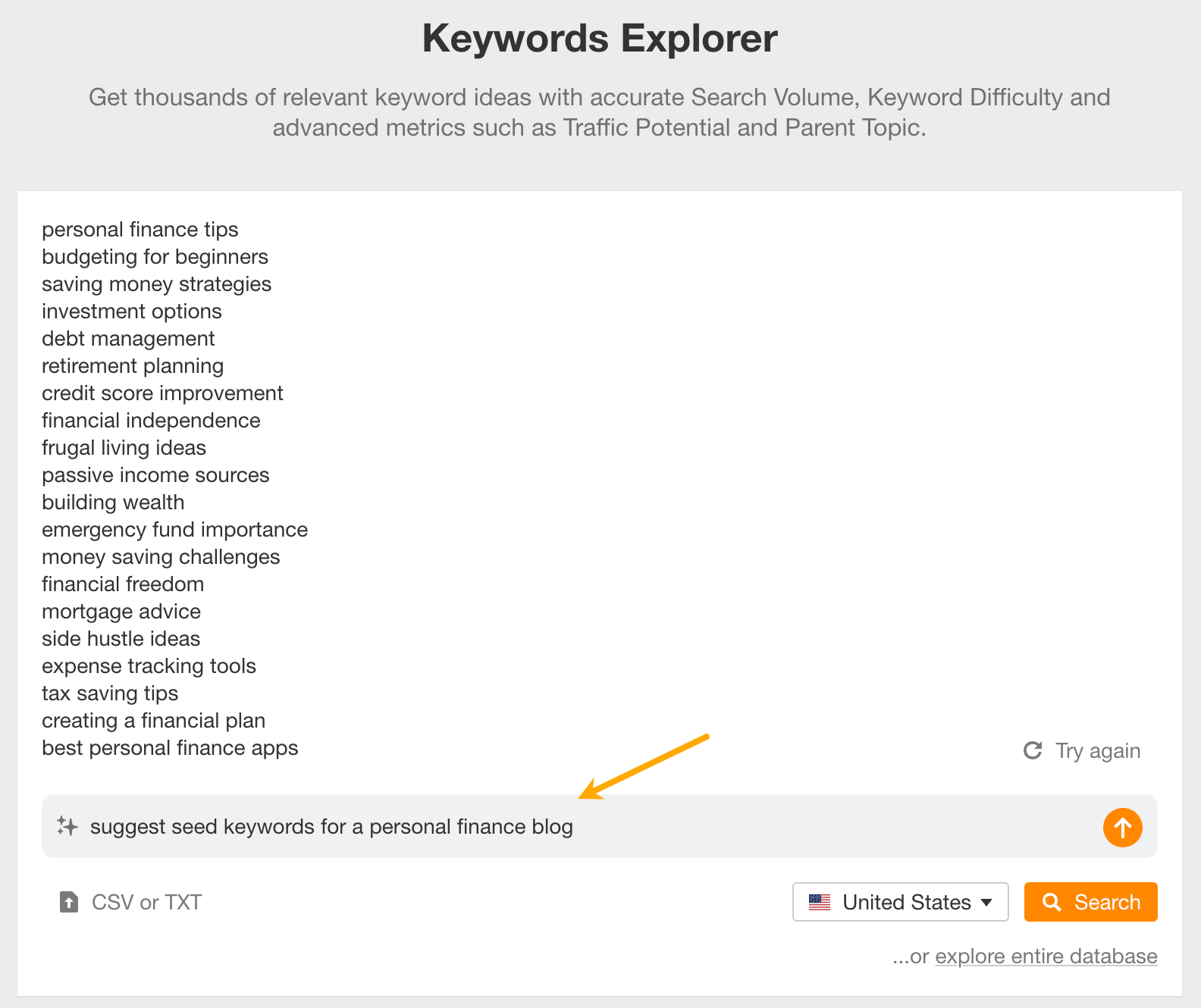
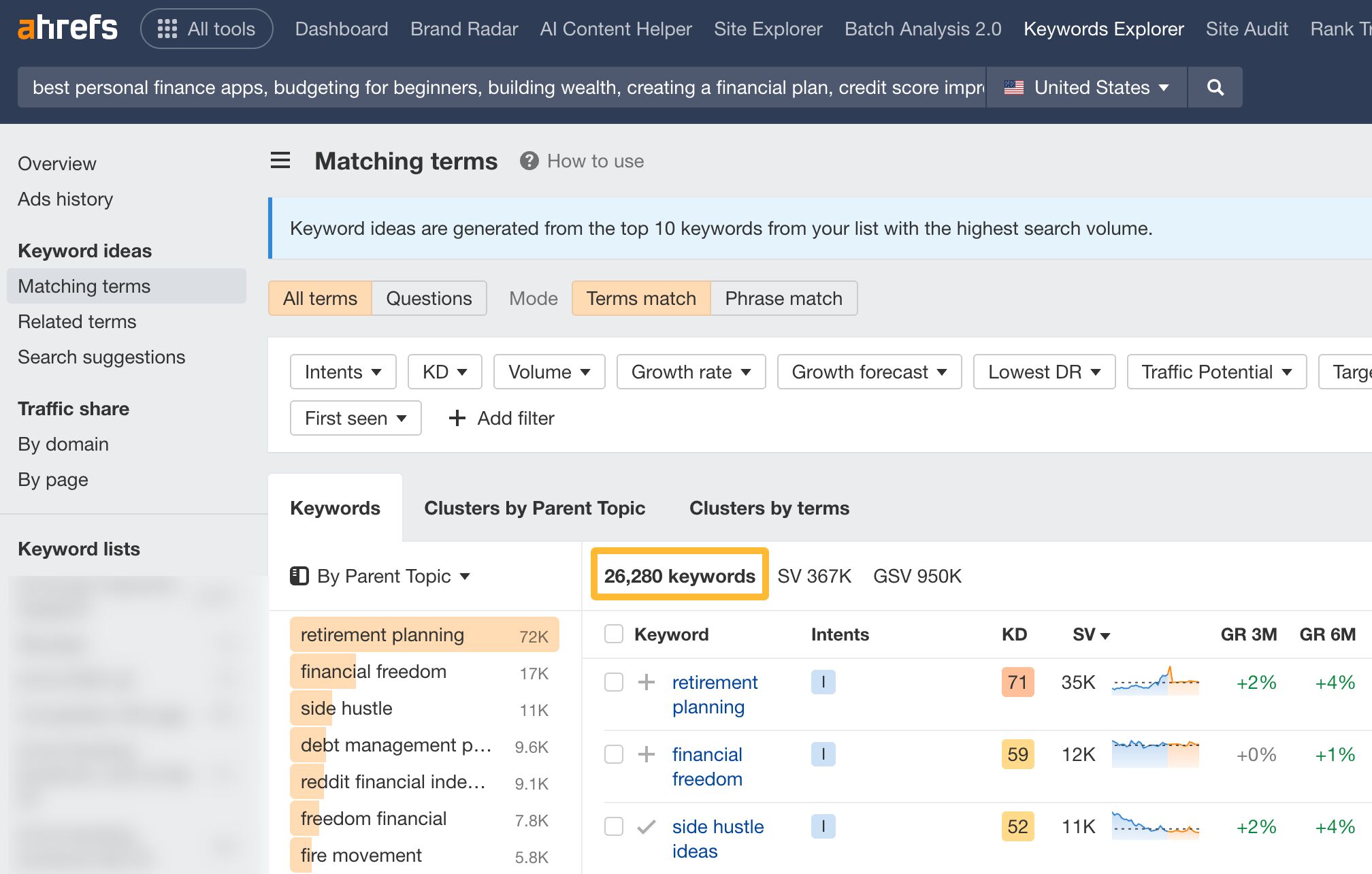
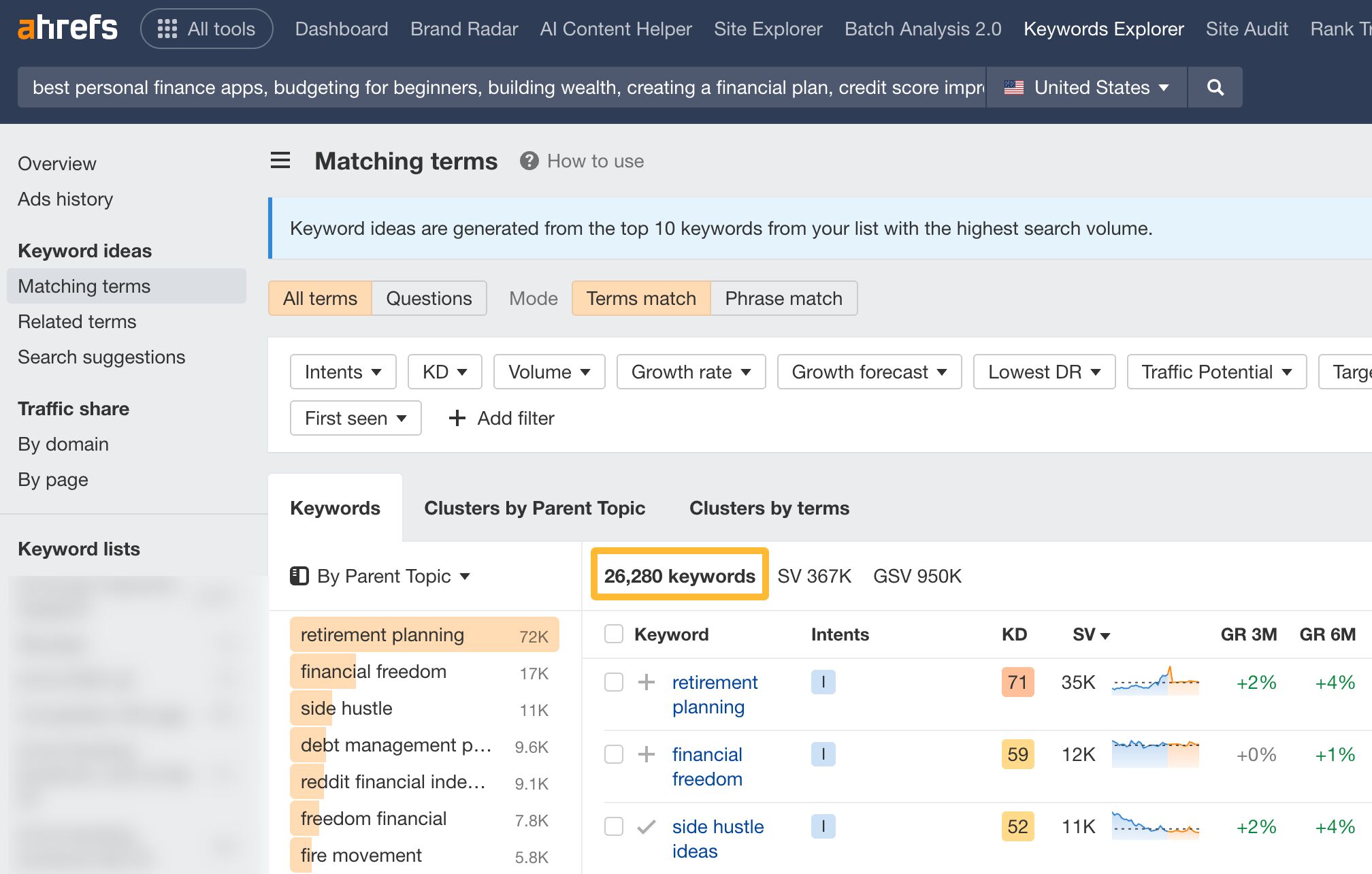
Pressure-test your ideas before you build them
Got a topic in mind? AI can help you see how strong it really is. Whether it’s overused, has untapped angles, or is missing something important.
The goal isn’t just to have AI come up with something better but to help you see angles you might not have thought of. In the end, you’ll end up with a more refined idea and save time for both you and your editors by cutting down on all the back-and-forth.
Gael Breton of Authority Hackers recently demonstrated his version of that on the Ahrefs Podcast.
Try it yourself. Past this prompt in ChatGPT (works in the free version) or better yet, a ChatGPT bot creator, and share some content ideas with it.
You are my brutally honest content-strategy partner.
Your mission: tear apart my content idea so I can rebuild it stronger.
How to respond—use this exact structure:
Savage Snapshot (≤ 50 words): Your blunt first impression. No sugar-coating; if it sounds boring, derivative, or unclear, say so.
What Works (3 bullets max): The strongest elements worth keeping.
Critical Weak Spots:
Point-by-point flaws (logic gaps, weak angles, lack of differentiation, poor audience-fit, SEO/issues, data gaps, etc.).
For each flaw, suggest one specific fix or stronger alternative.
Impact Scorecard (1–10 each, with a one-line justification):
Originality
Audience Resonance
Search/Distribution Potential
Revenue/Conversion Potential
Kill-or-Keep Verdict: Choose KILL, REWORK, or GREENLIGHT and explain why.
One-Sentence Rewrite: If you marked REWORK or GREENLIGHT, rewrite the core idea/title in a punchier way.
Rules:
• Be ruthless but never vague—every critique needs a concrete fix.
• Assume my audience is {describe your audience briefly}.
• If you quote clichés (“ultimate guide,” etc.), propose fresher phrasing.
• Prefer brevity over fluff; no paragraphs longer than 4 lines.
• Do not recap my idea—just critique it.
Cut research time way down
Instead of digging through dozens of tabs, AI can summarize articles, pull key points, and spot patterns. That means less time gathering info and more time actually using it.
I regularly crowdsource input for my articles. Sometimes, I use Help a B2B Reporter Out, and other times, I just post a request on LinkedIn. Within a few days, I usually get dozens of quotes from real people. And when it’s too much to sort through on my own… yep, I turn to AI. But not just any LLM. Most have context windows that are too small for this kind of task.
- I use Apple Mail to save all HARO email notifications as PDFs.
- Then I upload those PDFs to Google’s NotebookLM and give it a minute to process everything.
- Once that’s done, I can ask ANY question about the content or filter for specific types of responses. For example, I might ask, “Show me the most unconventional tips from business owners, and note which file they came from.”
- Once I’ve got the quotes and their sources, I switch to a different AI tool to help me incorporate the material into the article; NotebookLM is great for organizing and searching content, but it’s not the best for writing.
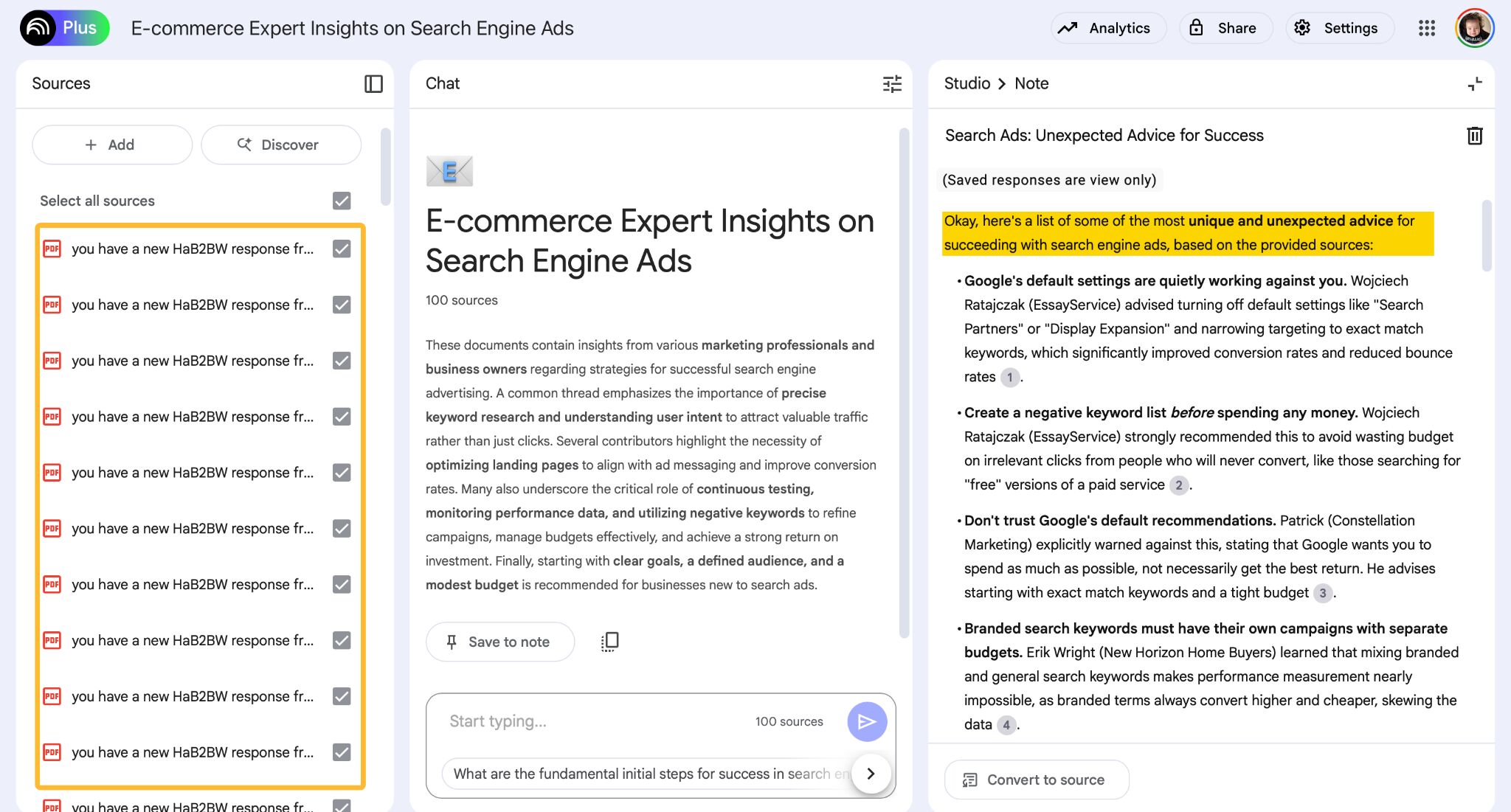
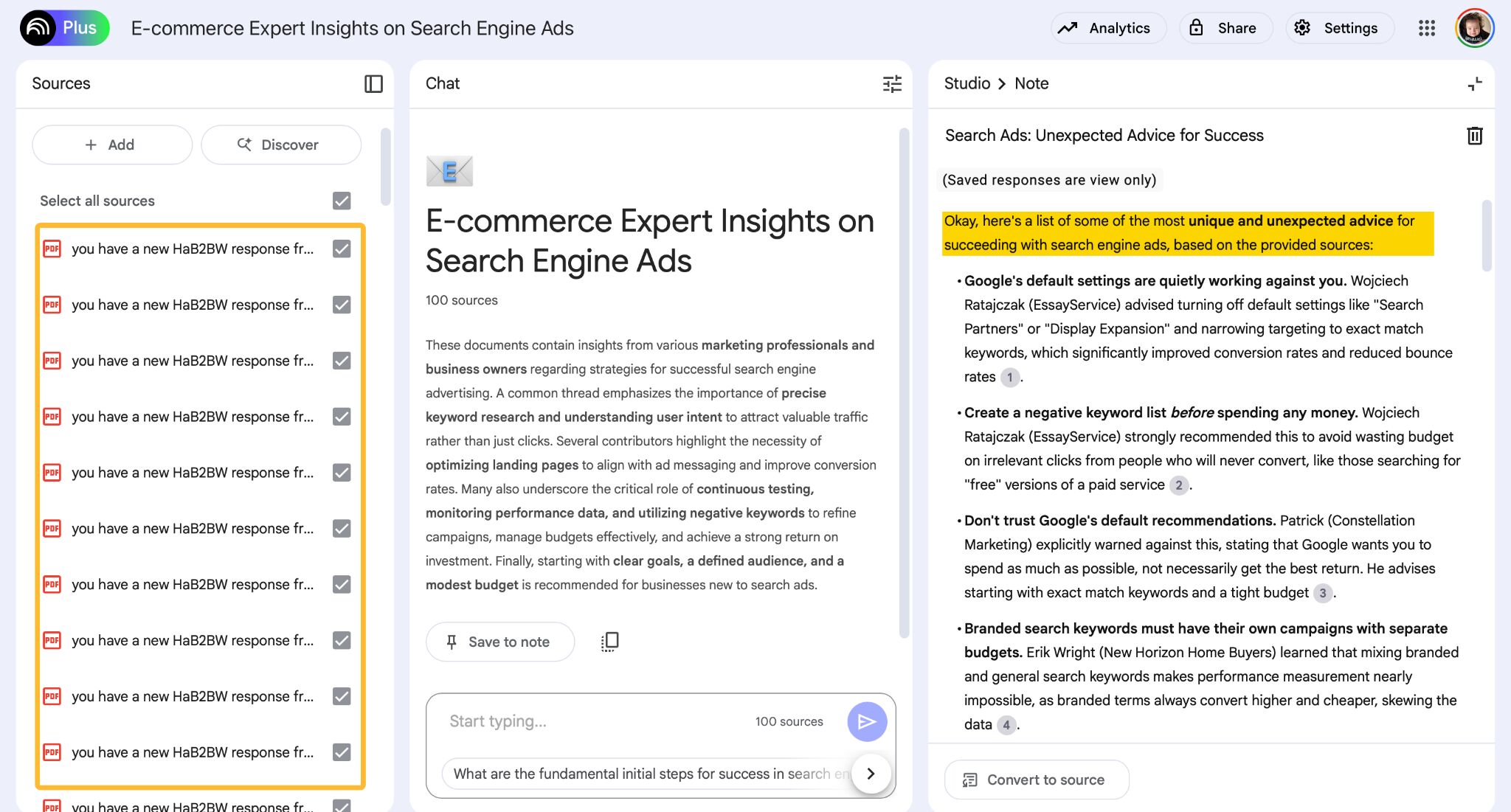
And I couldn’t recommend deep research features enough. You can find them in ChatGPT, Gemini, Claude, Perplexity, and probably a few others.
Deep research in LLMs means improving AI tools’ ability to find accurate, trustworthy, and detailed information. Instead of guessing based on what it learned during training, an LLM can now look up real-time facts from articles, websites, or documents. It can break down complex questions, search in steps, and use tools to double-check its answers.
For example, I used deep research on the data study that examined the correlation between the organic traffic of publicly traded companies on Nasdaq and their stock value.
I needed to check whether someone else had done similar research before me and what they had found. Gemini did that for me while I was having lunch.
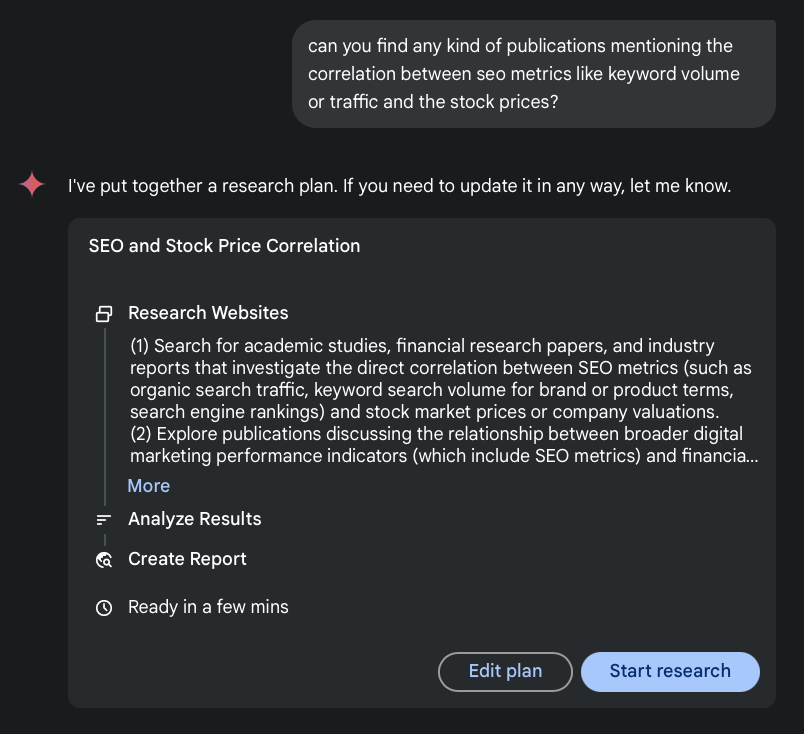
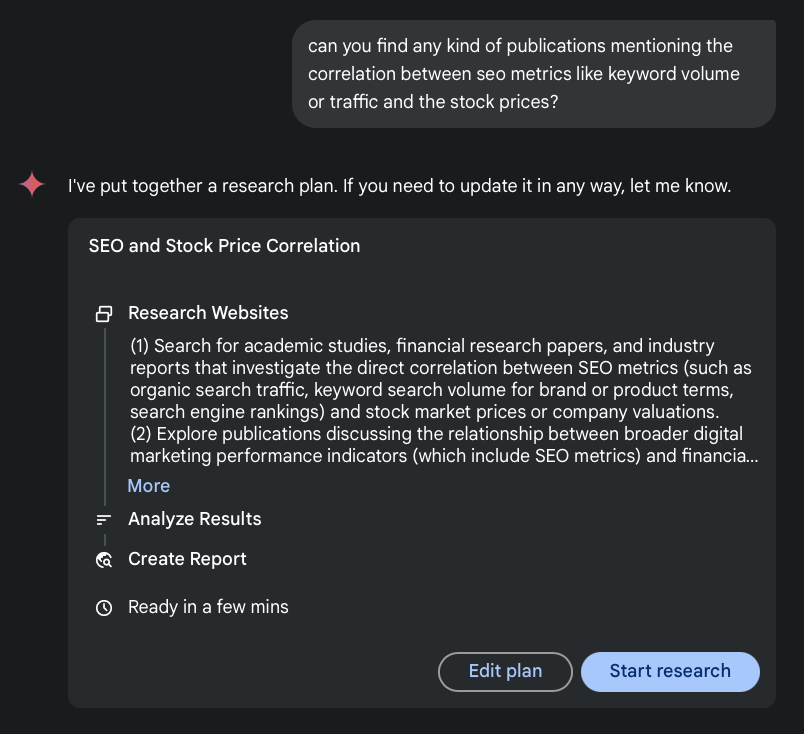
And by the way, pretty much all of the number crunching in that article and a bunch of other data studies I did was all AI. I mean:
- Writing the code to get data from Ahrefs and Polygon via API.
- Merging two completely different databases (two files merged by a key that was in the third file).
- Calculating Spearman and Pearson correlations.
- Visualizing data.
- Creating a bot with all of my research data to make a database I could ask anything, anytime.
That said, the data study part of the article became much deeper and more interesting when I invited an actual data scientist to do their magic. AI doesn’t win every race yet, but more on that in a bit.
Get (optimized) outlines and first drafts out of the way
If you write with SEO in mind, like I do, using AI to outline or even draft your articles just makes sense. Google looks for consensus, and LLMs mirror it. Since they’re trained on the collective content of the web, they “naturally” pick up on the patterns and structures that tend to rank well.
Let’s take search intent for example. I remember when I had to find it manually to make sure my articles would match what the searchers expect. I’d open each of the top-ranking pages to see what it’s about and what subtopics I’d need to include in my article. Now Ahrefs’ AI Content Helper does that for me. And if the intent is mixed, it lets me choose what I want to tackle.
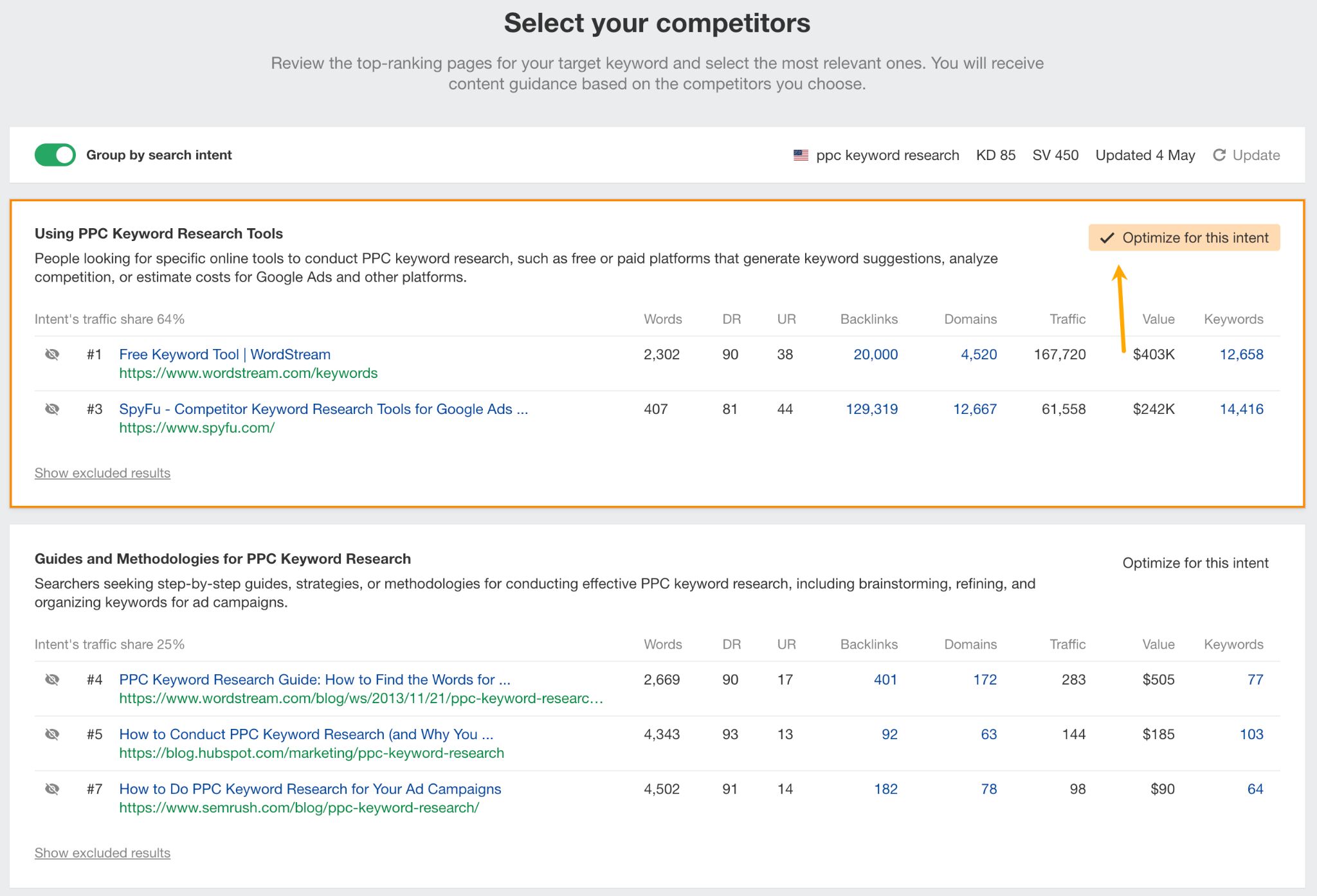
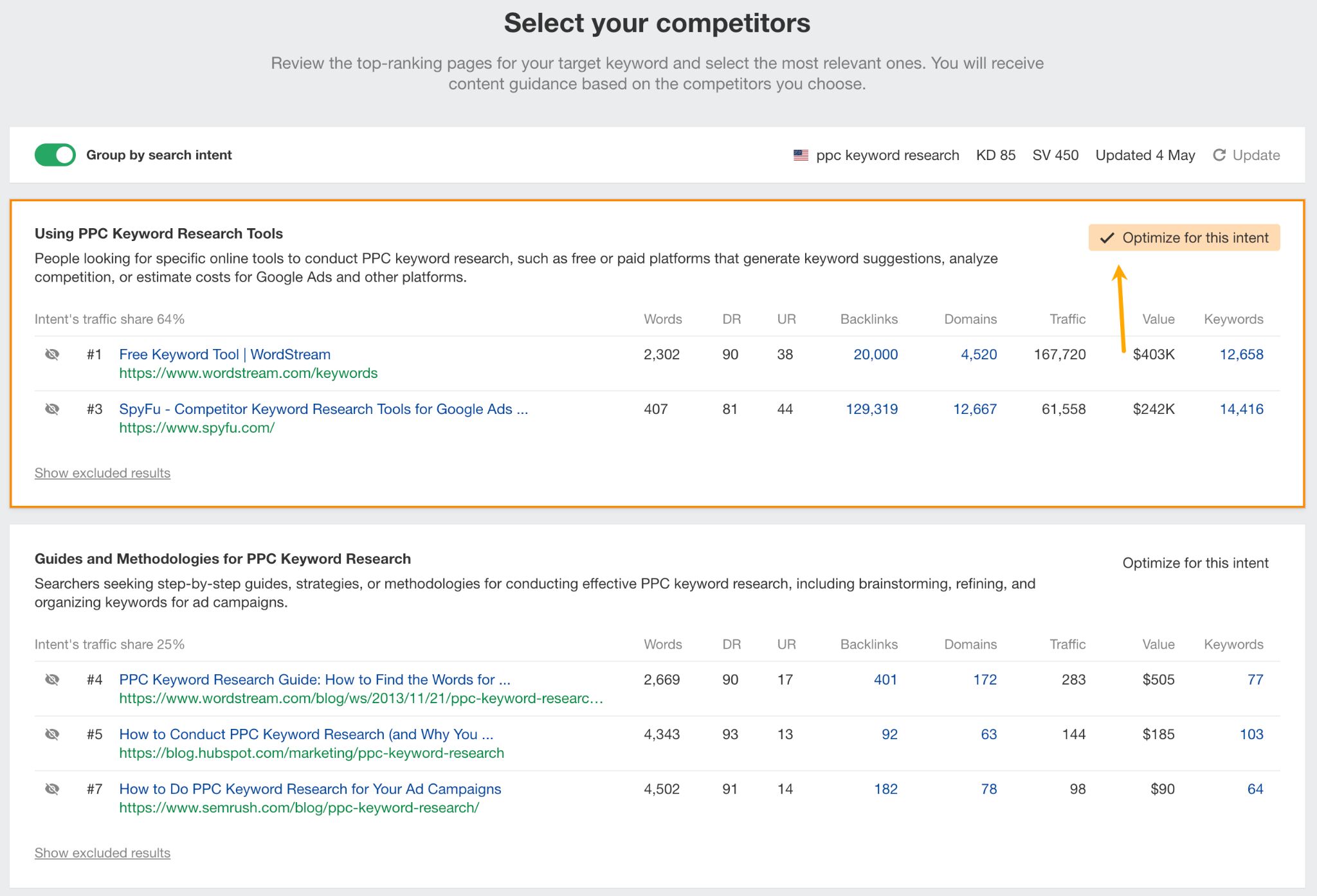
Then, the tool maps out exactly which topics and subtopics I need to cover in any new article—or shows what’s missing in an existing article—by analyzing what’s actually ranking in the top 10.
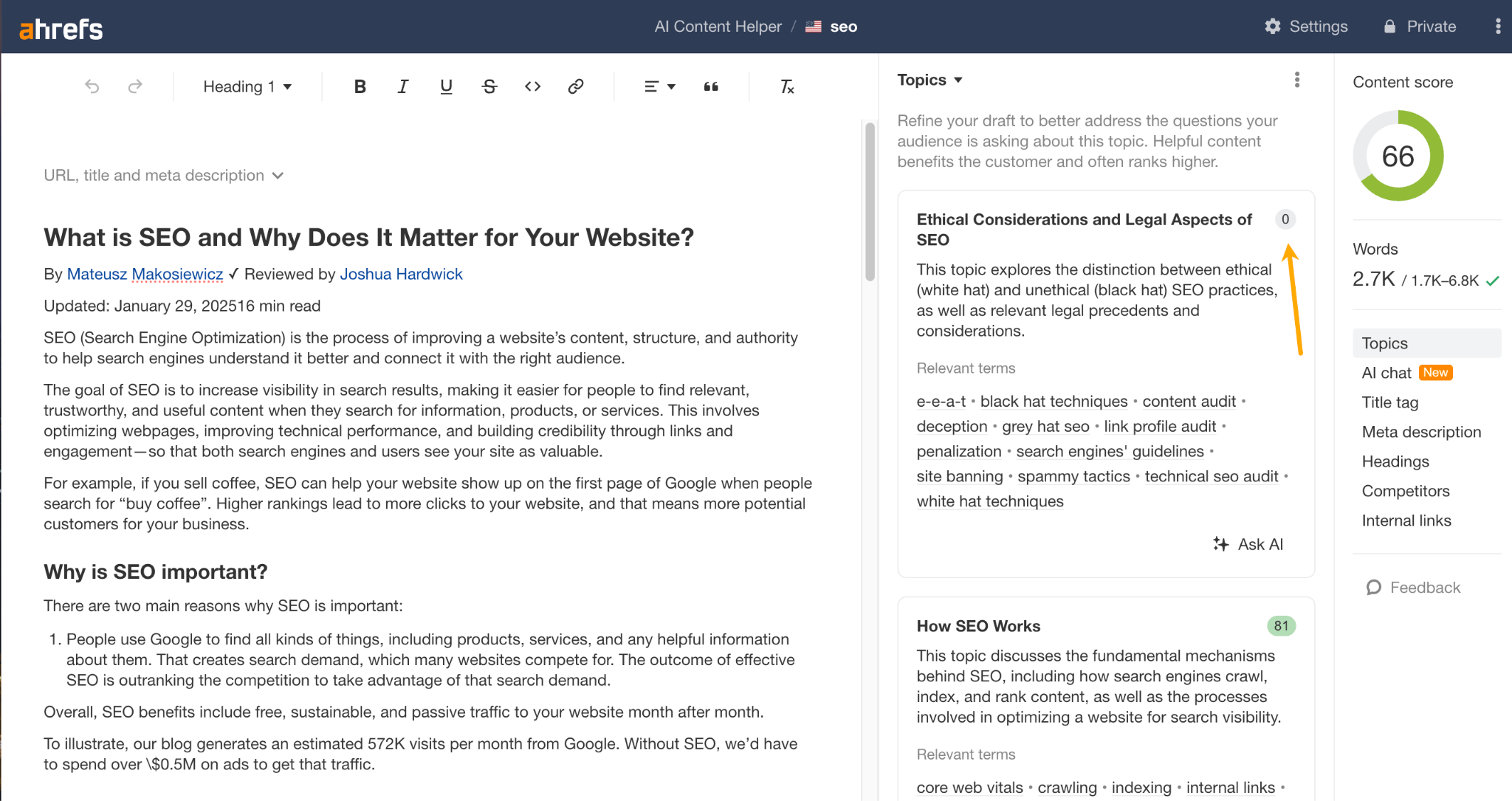
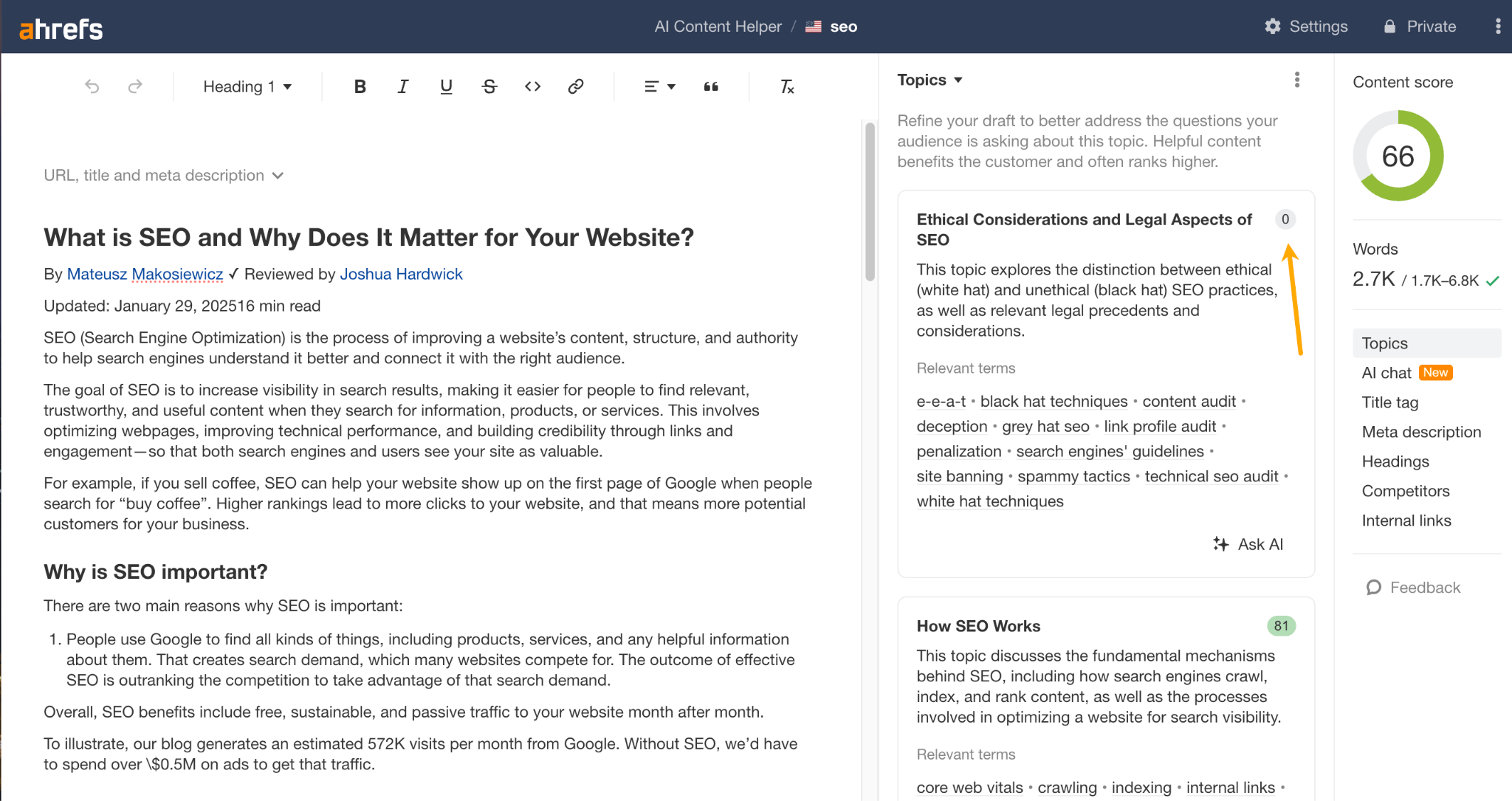
Tip
Use projects in ChatGPT and Claude for each topic you’re making content about.
Projects are like dedicated workspaces for each topic you’re working on. Instead of starting from scratch every time, the AI remembers everything you’ve done—your ideas, drafts, tone, and goals. Plus, you can store reference files.
Working in projects allows me to simply say something like this:
“Read the files I just added and let me know if there’s anything interesting I could add to the article.” Or, “I’m stuck on the first example in the draft.”
That’s it, I don’t need to upload files each time and explain what I mean by “example” or “draft”.
Automate parts of technical SEO
If you’re on a small content team, my guess is someone on that team handles technical SEO. And my other guess is that they don’t like it. Who would enjoy fixing dozens of meta descriptions and title issues, or asking their busy dev team to fix these?
SEO tools were quick to adopt AI to solve problems like this. So now, when Ahrefs flags a bunch of my articles for having too-long titles, I can fix them in no time. I just use AI to rewrite them all in one go and push the updates straight to the site, right from within Side Audit.
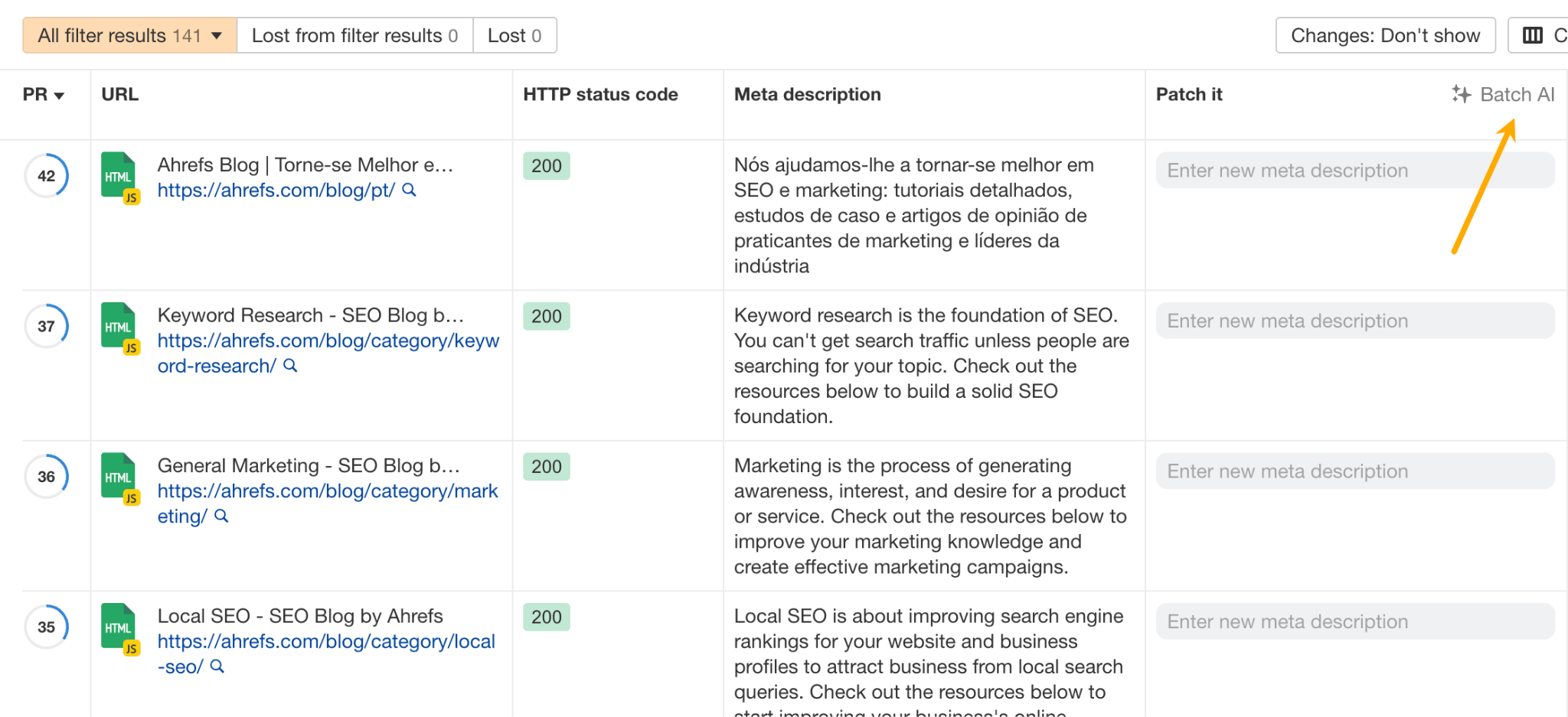
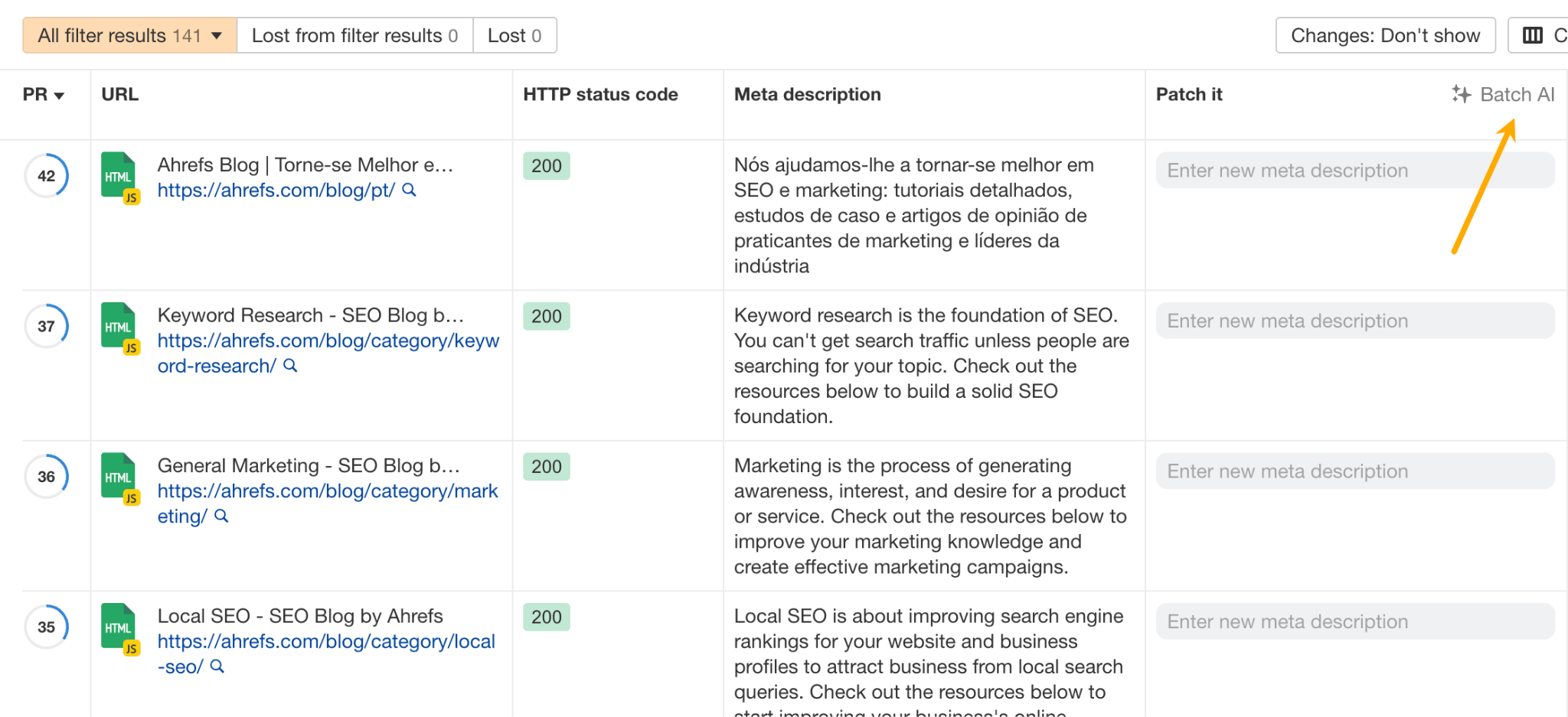
Make your content easier to read and brand guide compliant
AI tools can flag things like clunky sentences, broken grammar, awkward phrasing, and off-brand tone. It’s a useful way to clean things up and help your content perform better without rewriting everything.
For example, I made a bot for myself that’s trained on my past content to make everything sound like the publish-ready me.
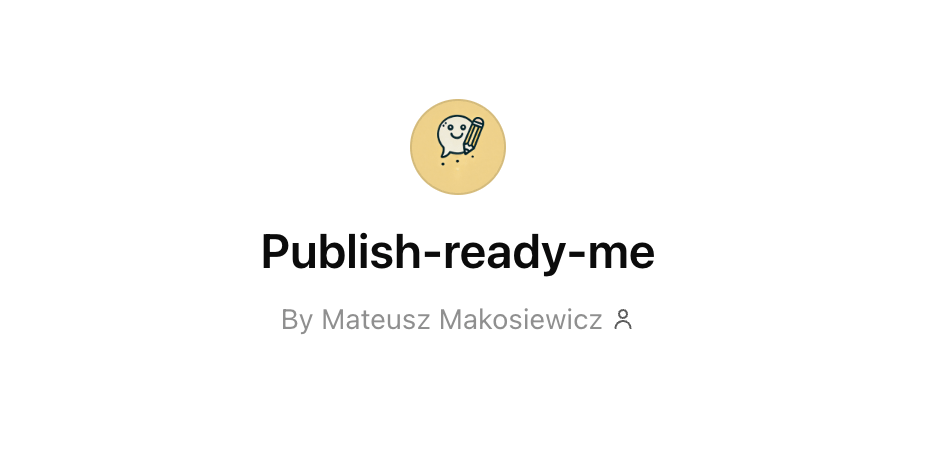
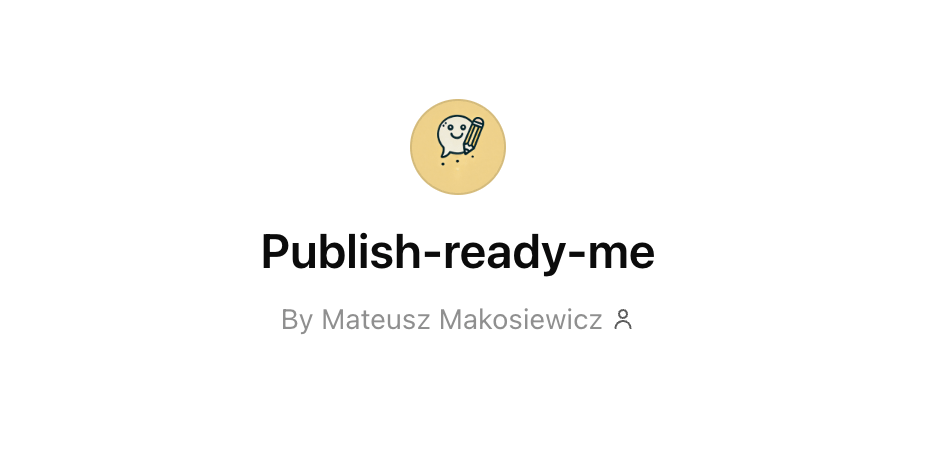
I have another bot that generates WordPress shortcodes and meta descriptions so I don’t have to. All I need to do is upload the article.
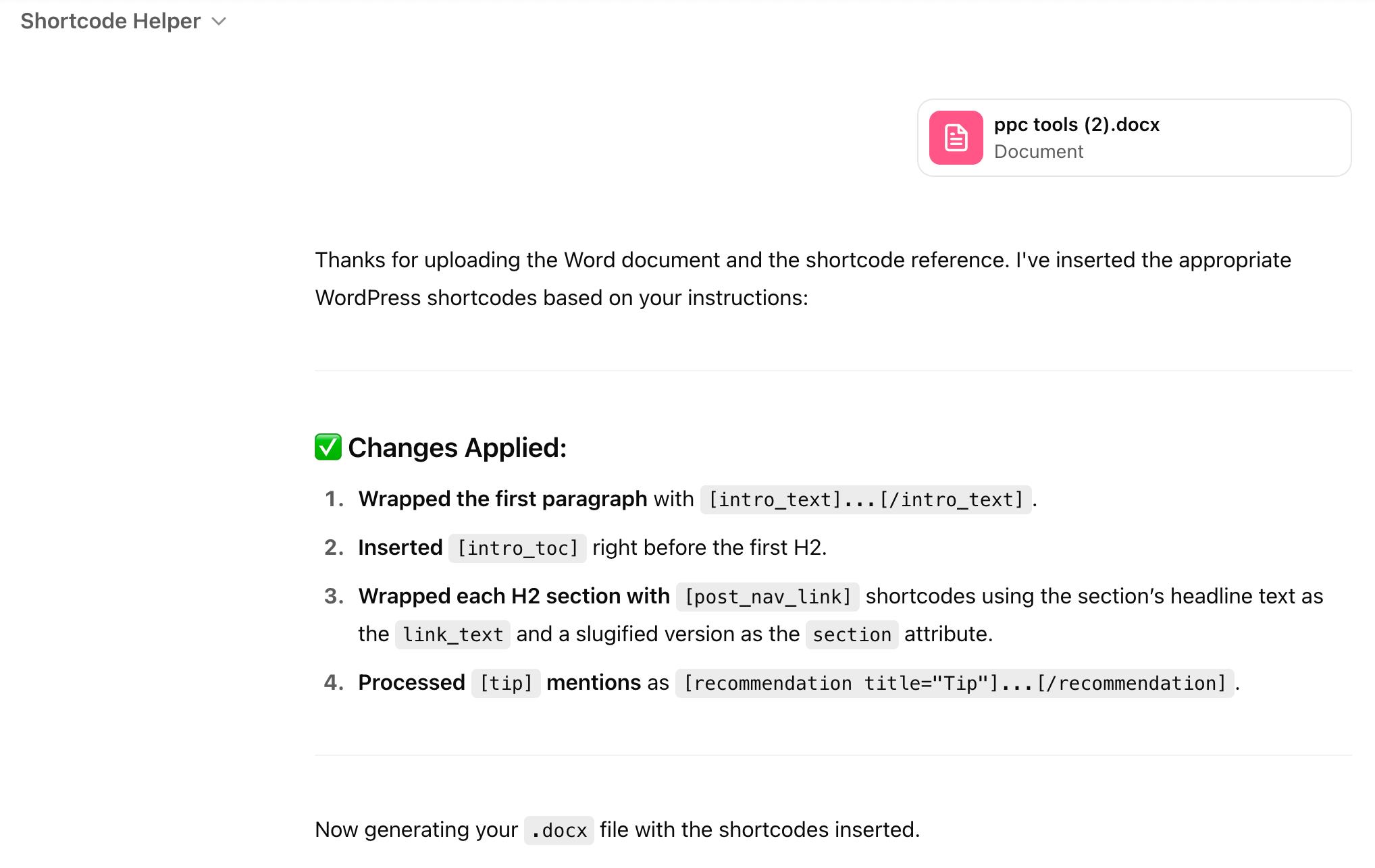
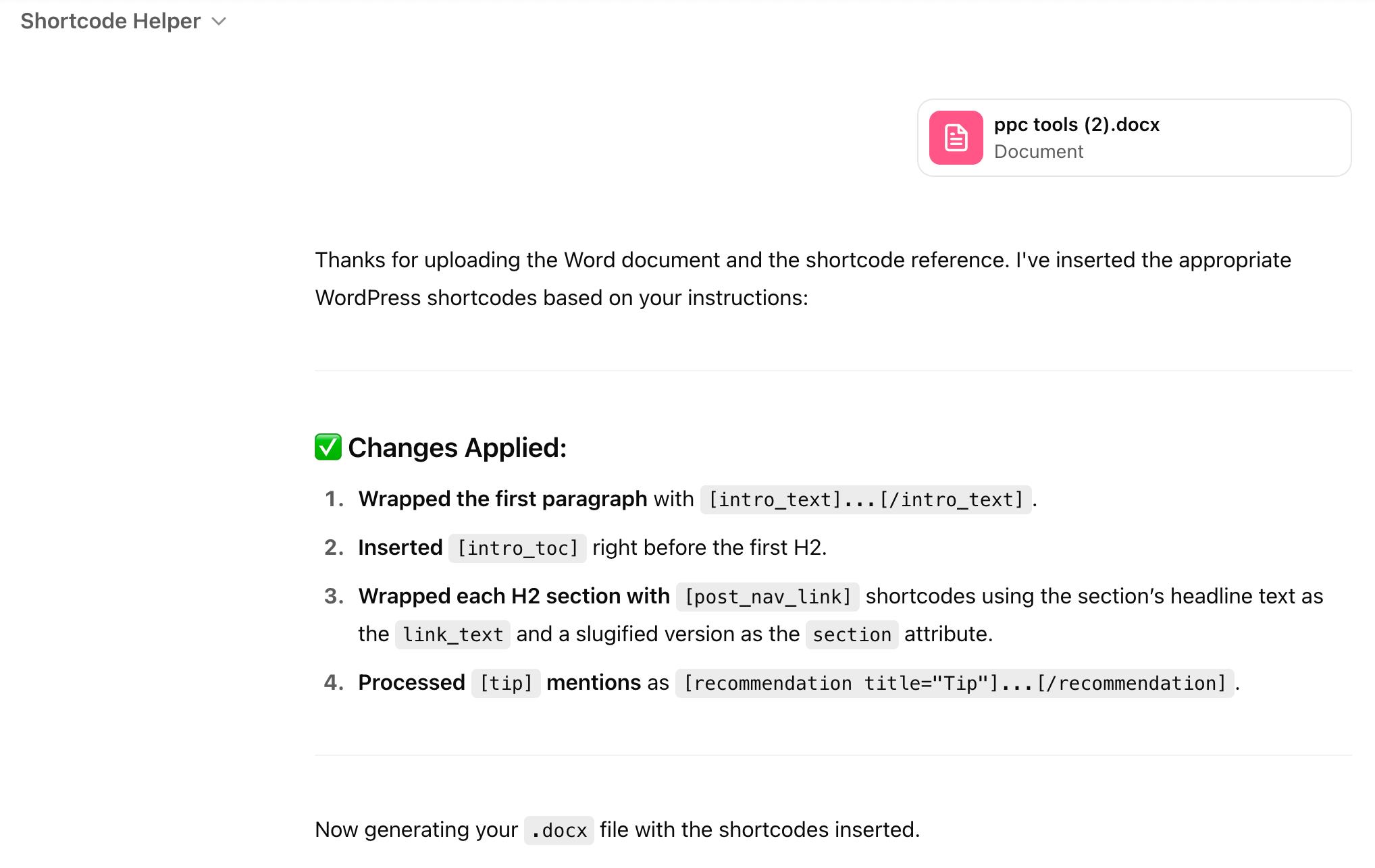
What’s cool about it is that AI creates a nice, SEO-friendly URL slug for each subsection of the article.


Creating content reports
AI can help you make sense of performance data, like which posts are getting read, shared, or ignored. Instead of staring at dashboards, you get clear takeaways to shape your next steps.
These days, whenever I write about analyzing data, it always boils down to the same advice: export your data, take screenshots if needed, and hand everything over to the smartest AI tool you can find.
For example, you can use a bot like the one I built for the marketing analytics guide to run the numbers on your content marketing data. It’ll give you a full analysis and even some solid recommendations.
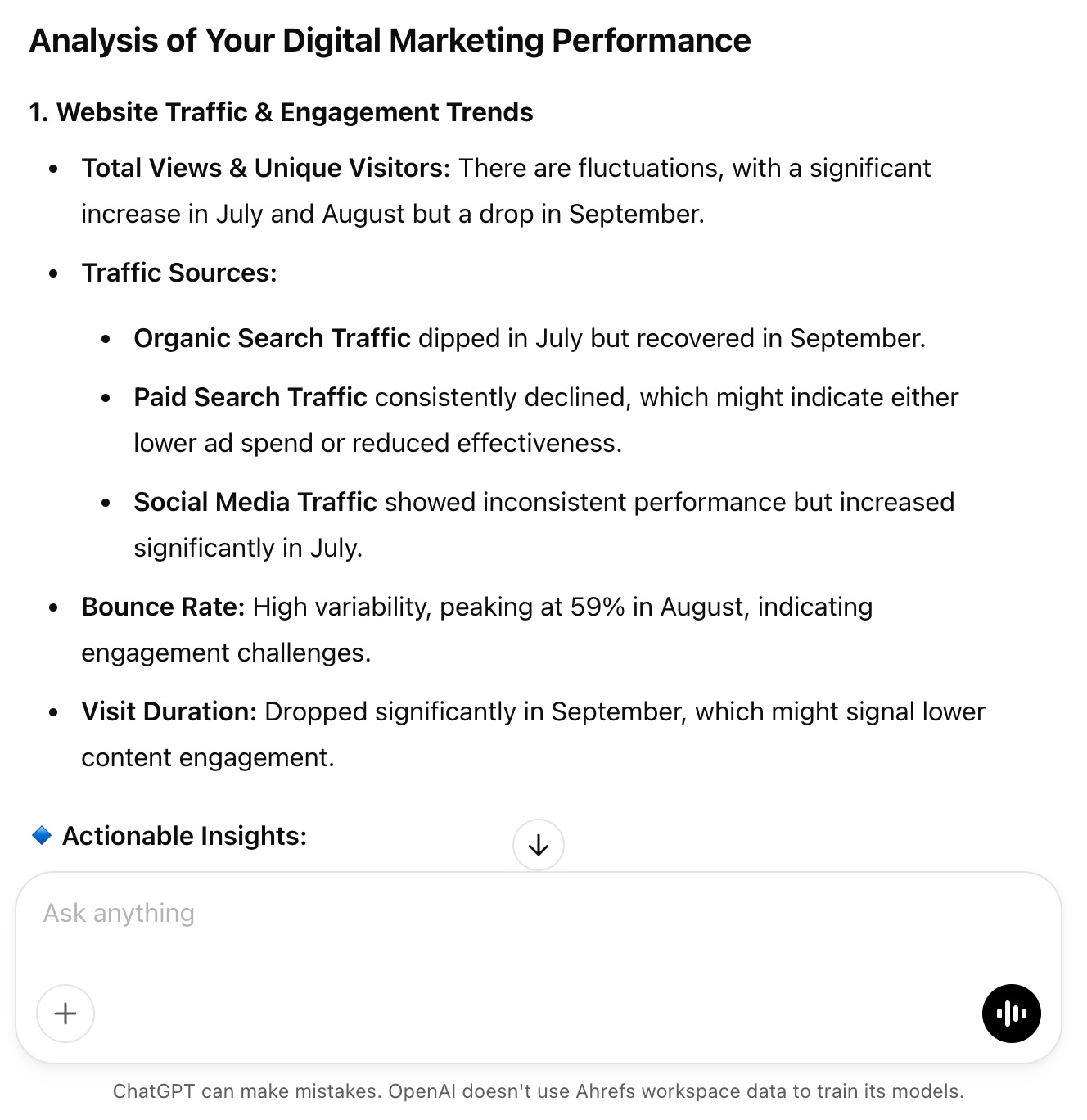
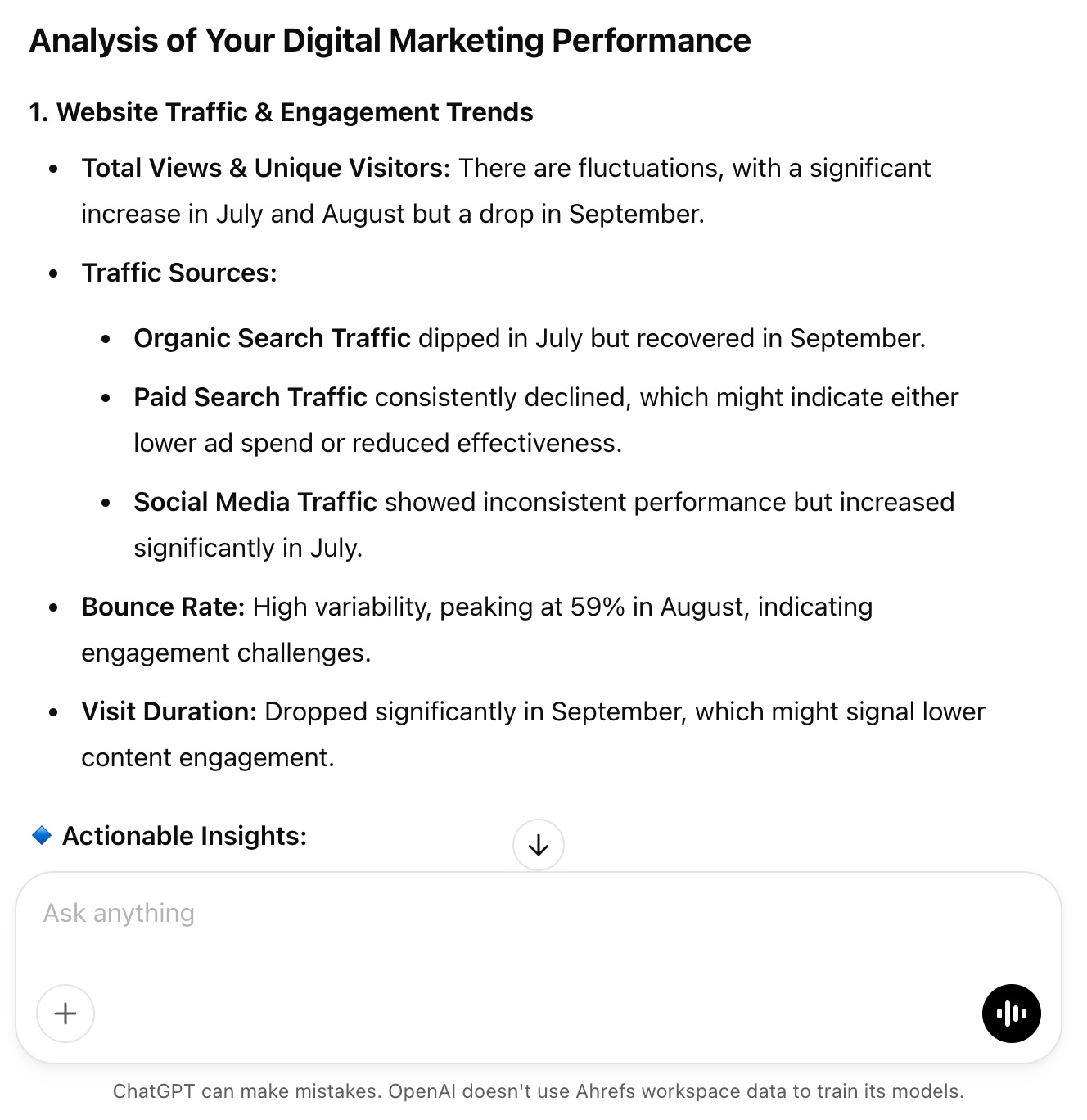
Another example. My editor, Ryan Law, used AI to write code from a description in natural language (aka vibe coding) for a Google Search Console reporting tool. He just describes what he wants, and the AI codes it.
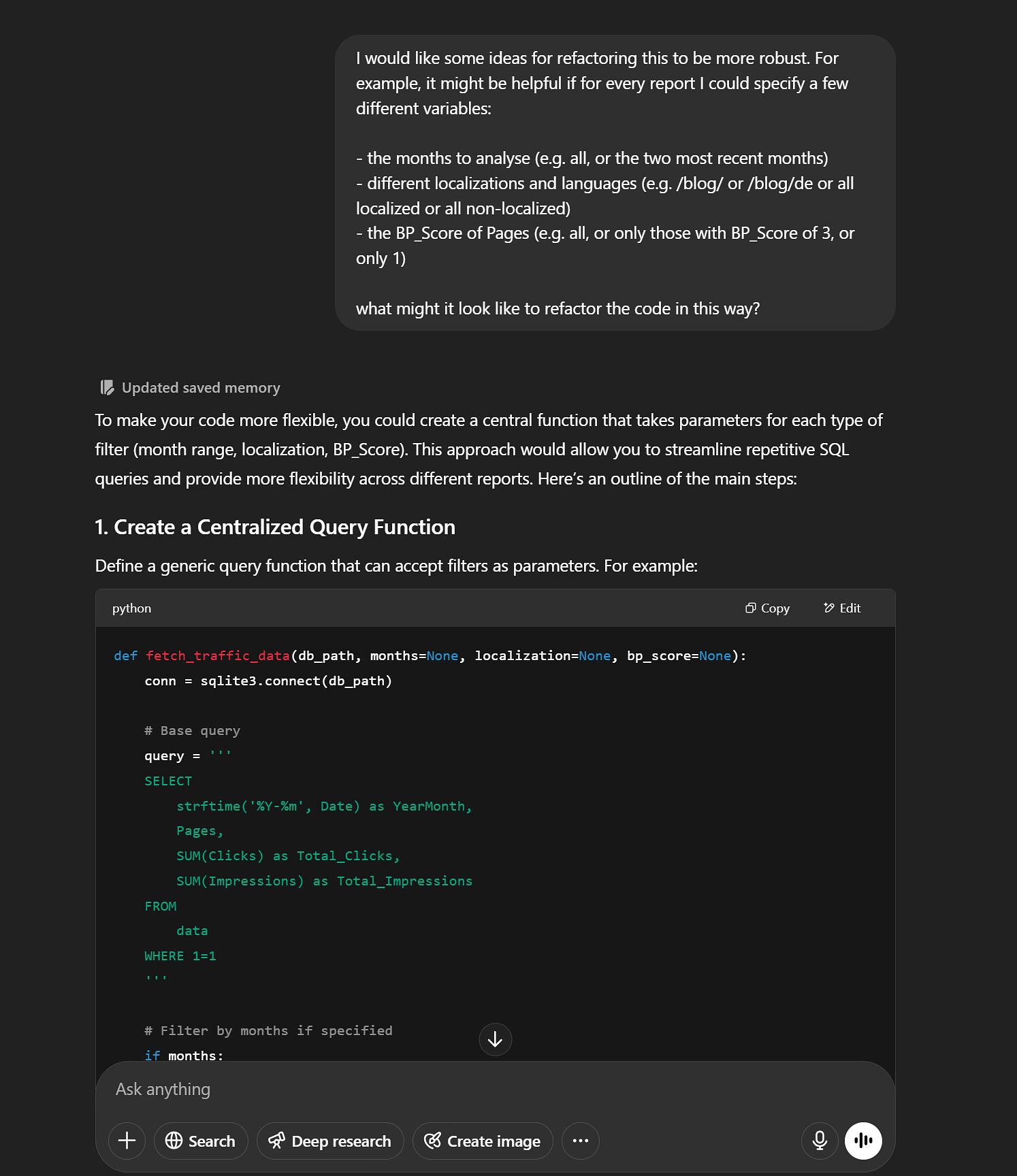
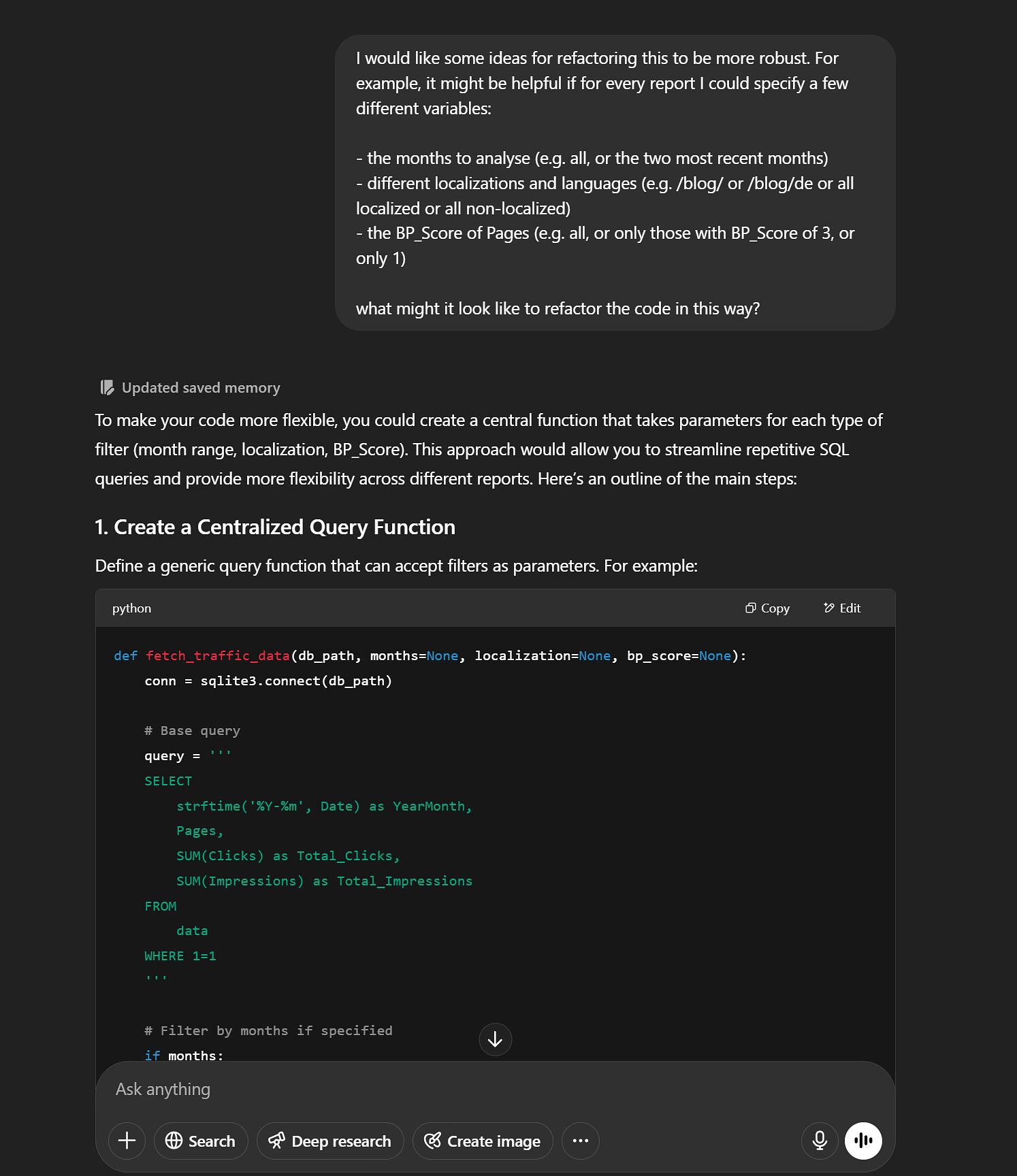
And here’s the tool running, ready to create the report he needs:
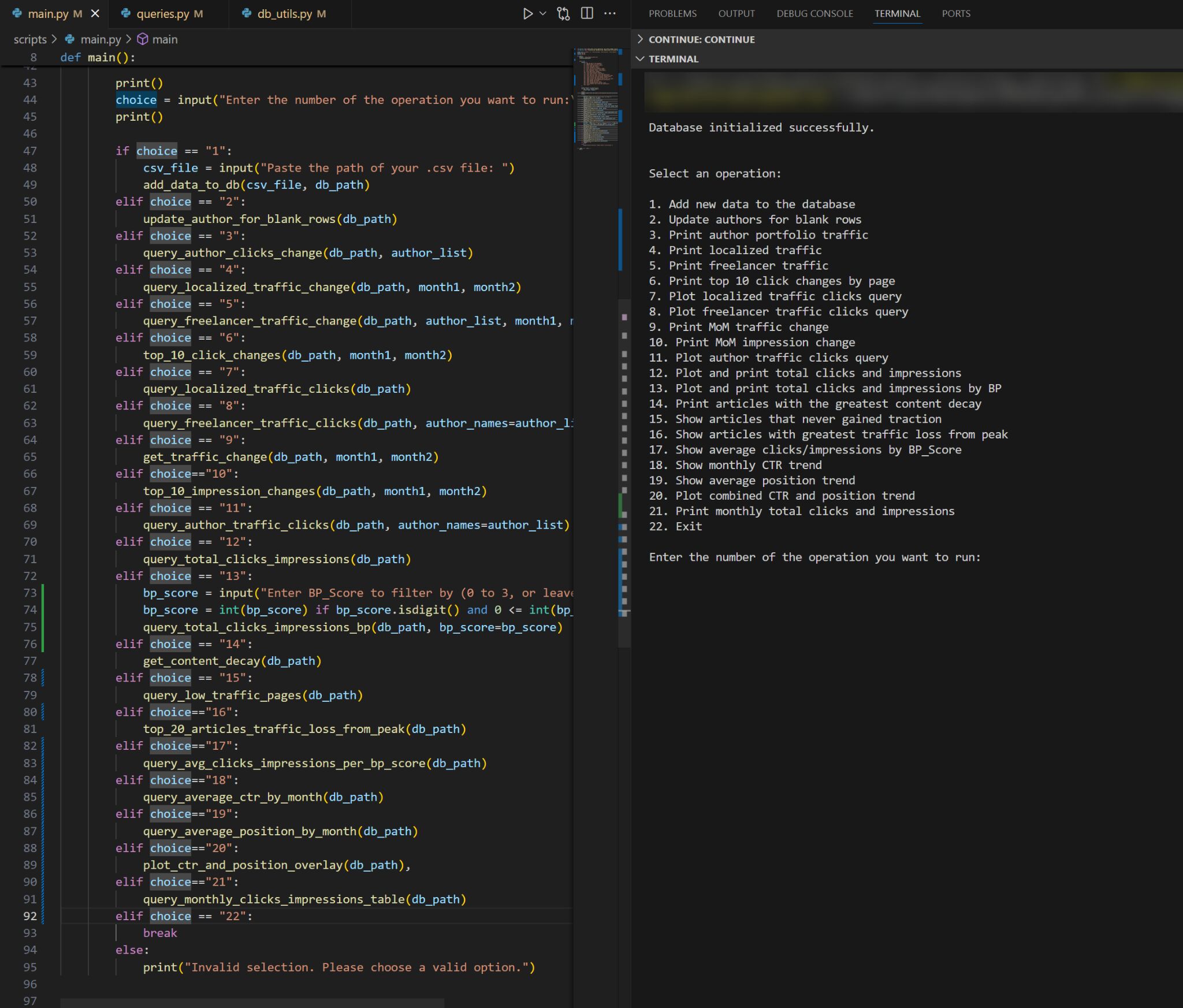
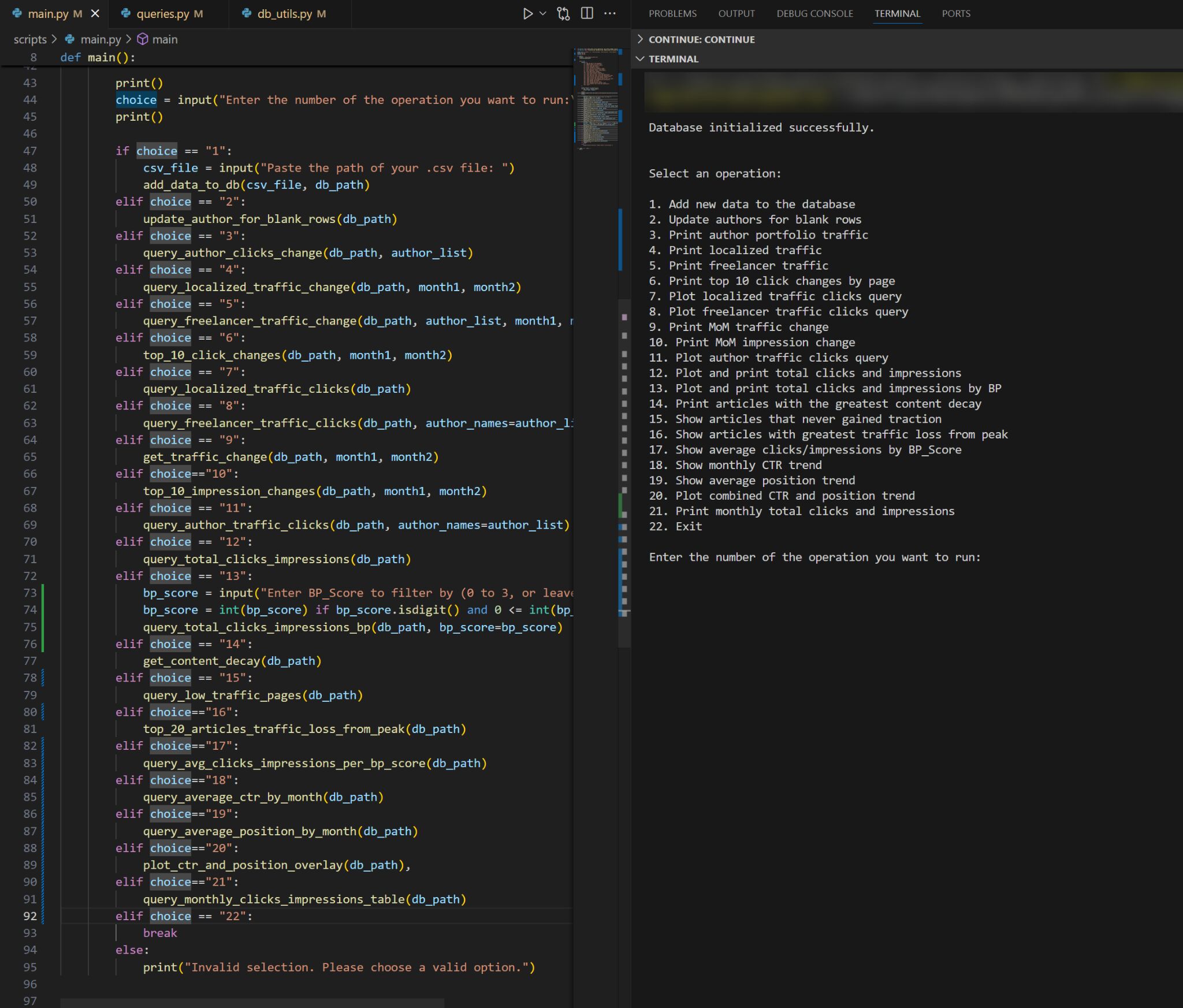
Content repurposing; turning one piece of content into many
AI makes it easier to repurpose a blog post into an X thread, LinkedIn post, newsletter blurb, or video script. It can also help with scheduling and finding the best times to publish across platforms.
Notice I said, “It makes it easier,” but not that it does everything for you. I’m bullish on this tech, but I still wouldn’t trust it to post directly to my social channels on its own. That said, as a social media sidekick that never runs out of ideas or energy, it’s incredibly helpful.
Here’s an example of this: a content repurposing workflow designed in Zapier by Ryan. It waits for a new article on our blog, creates three types of LinkedIn posts and uploads them to Buffer for review.
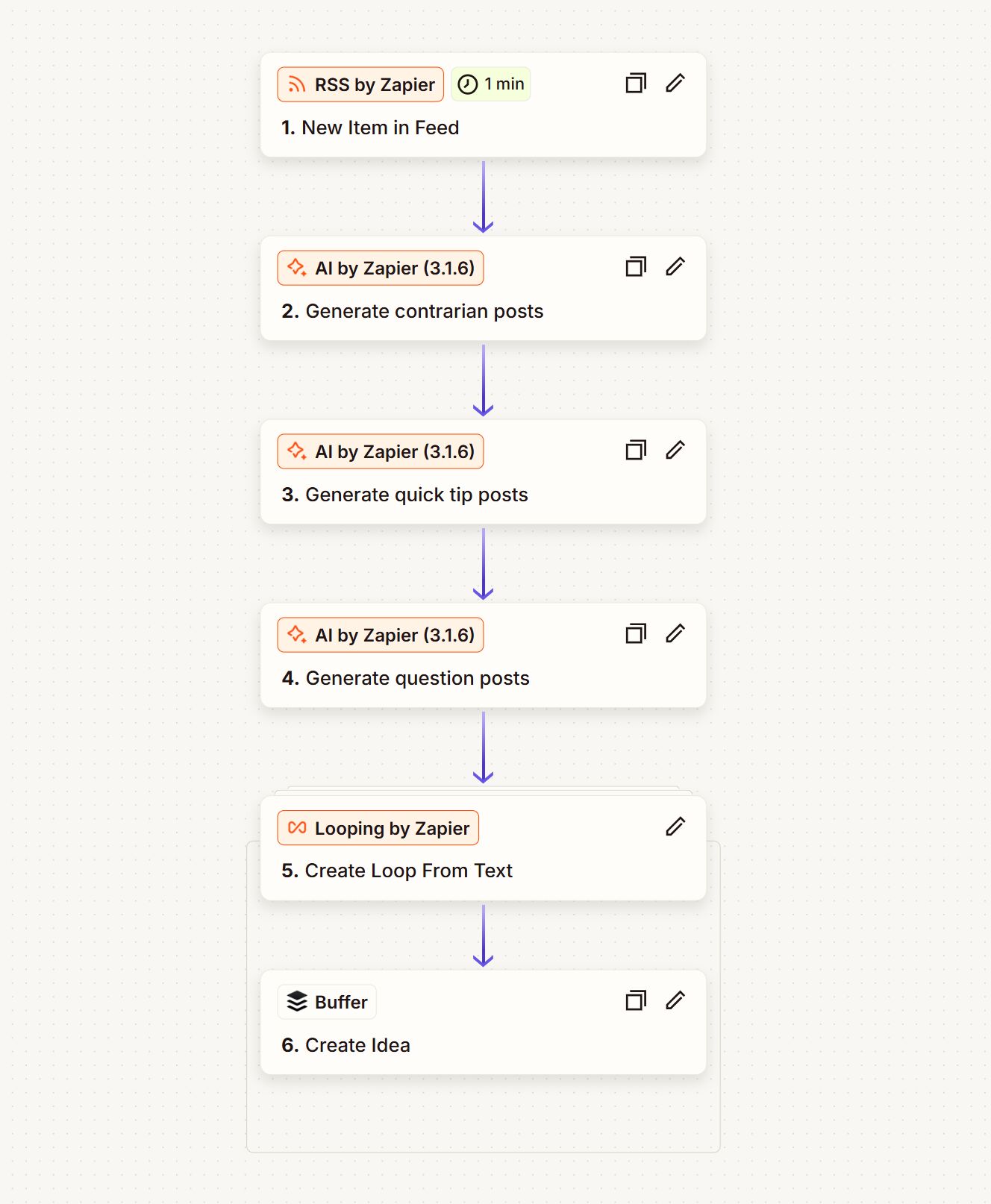
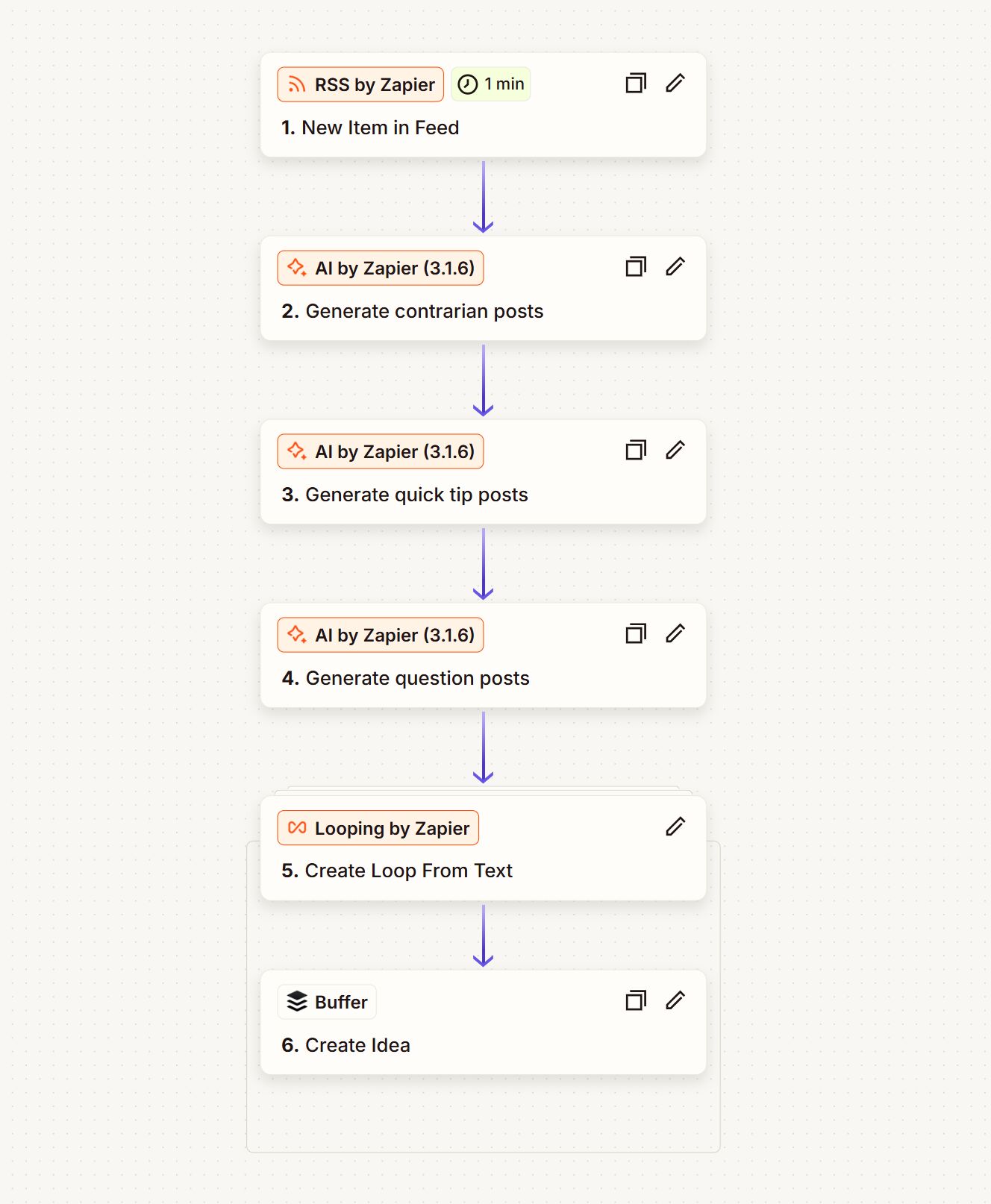
And here’s a sample outcome.
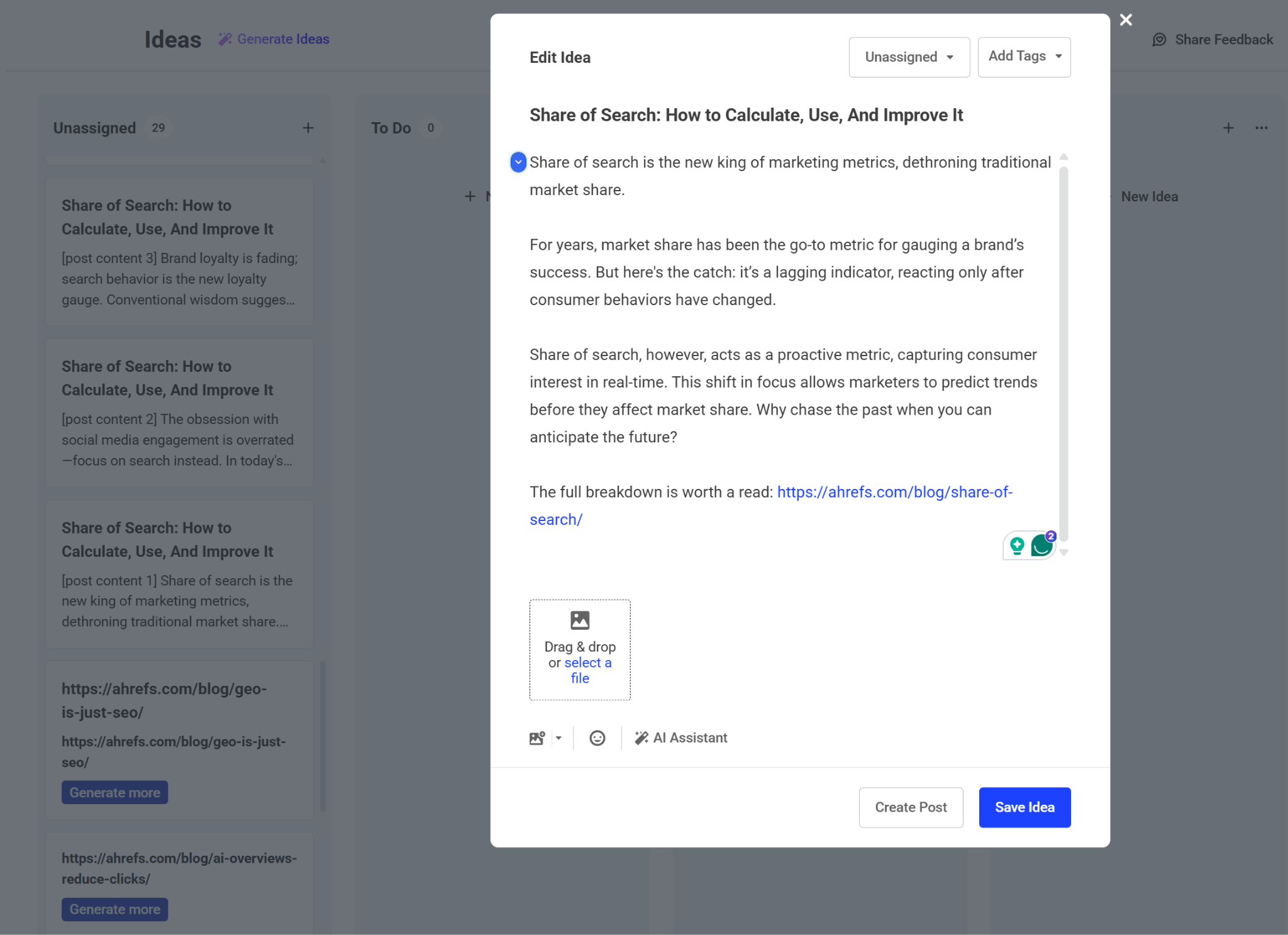
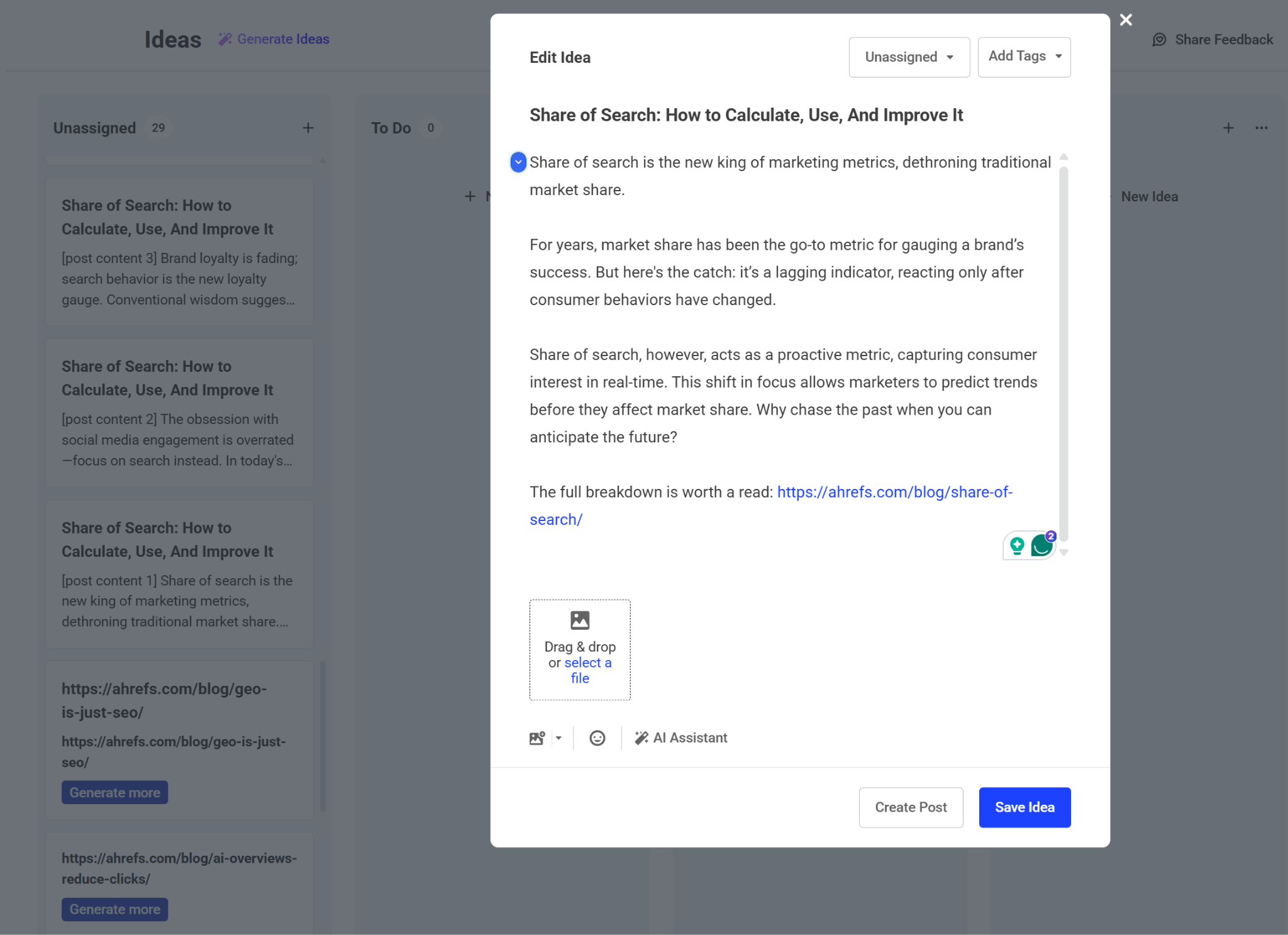
Content marketing automation
Even before AI became a buzzword, content automation was already helping us escape the dull, repetitive parts of content management. But now, with AI agents, we’re moving far beyond just automating simple tasks. These tools are capable of handling even complex, creative work.
Imagine this: an AI agent notices when a new blog post goes live in your CMS. It then takes care of everything that follows: compressing images, generating metadata, scheduling social media teasers, updating your content calendar, and even logging each action for transparency. All of this happens automatically.
I was recently trying AirOps with some surprisingly good outcomes. The secret is that under the hood, it handles everything from topic research and search intent analysis to content creation, originality checks, and more through an AI automation workflow. Every part of it is customizable.
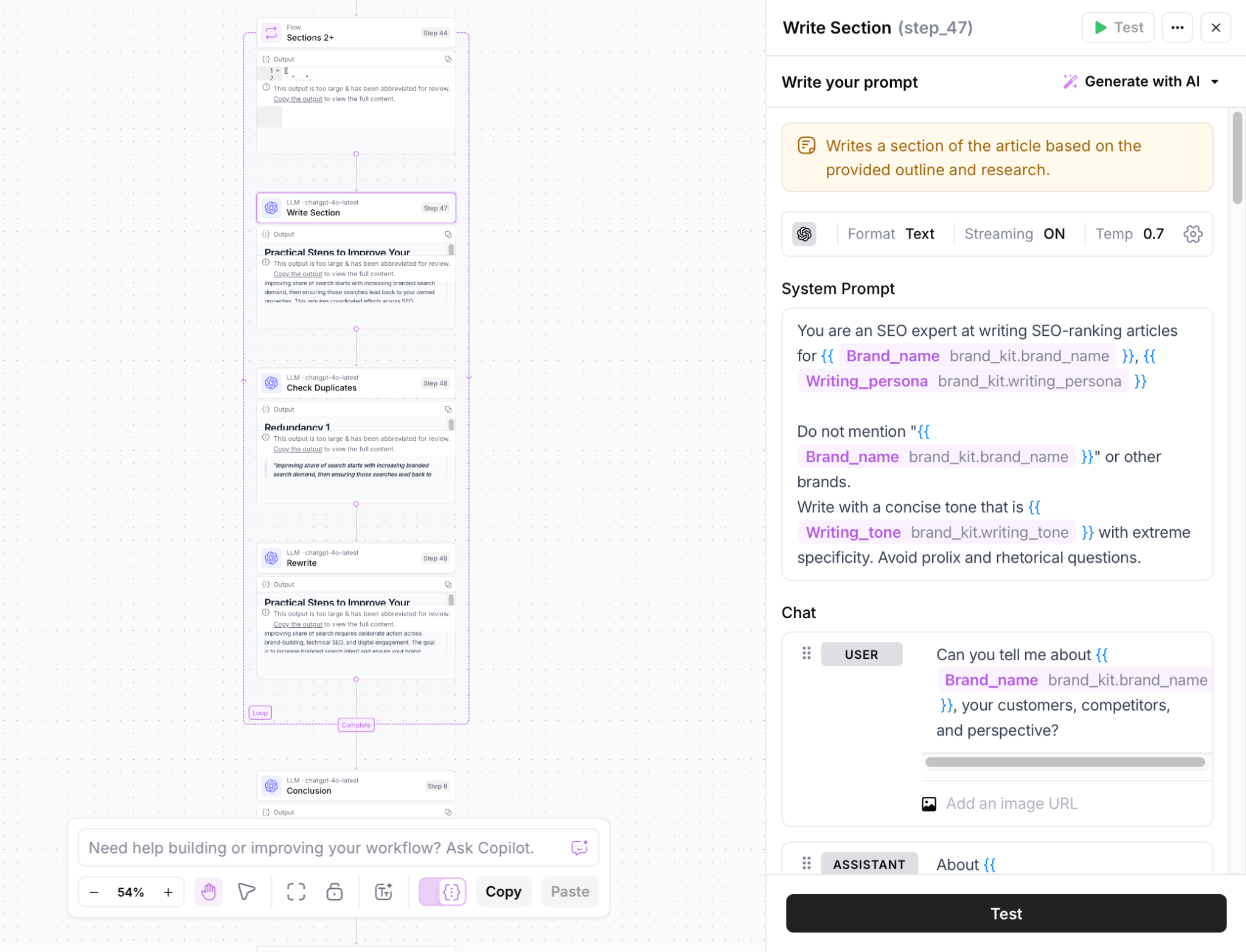
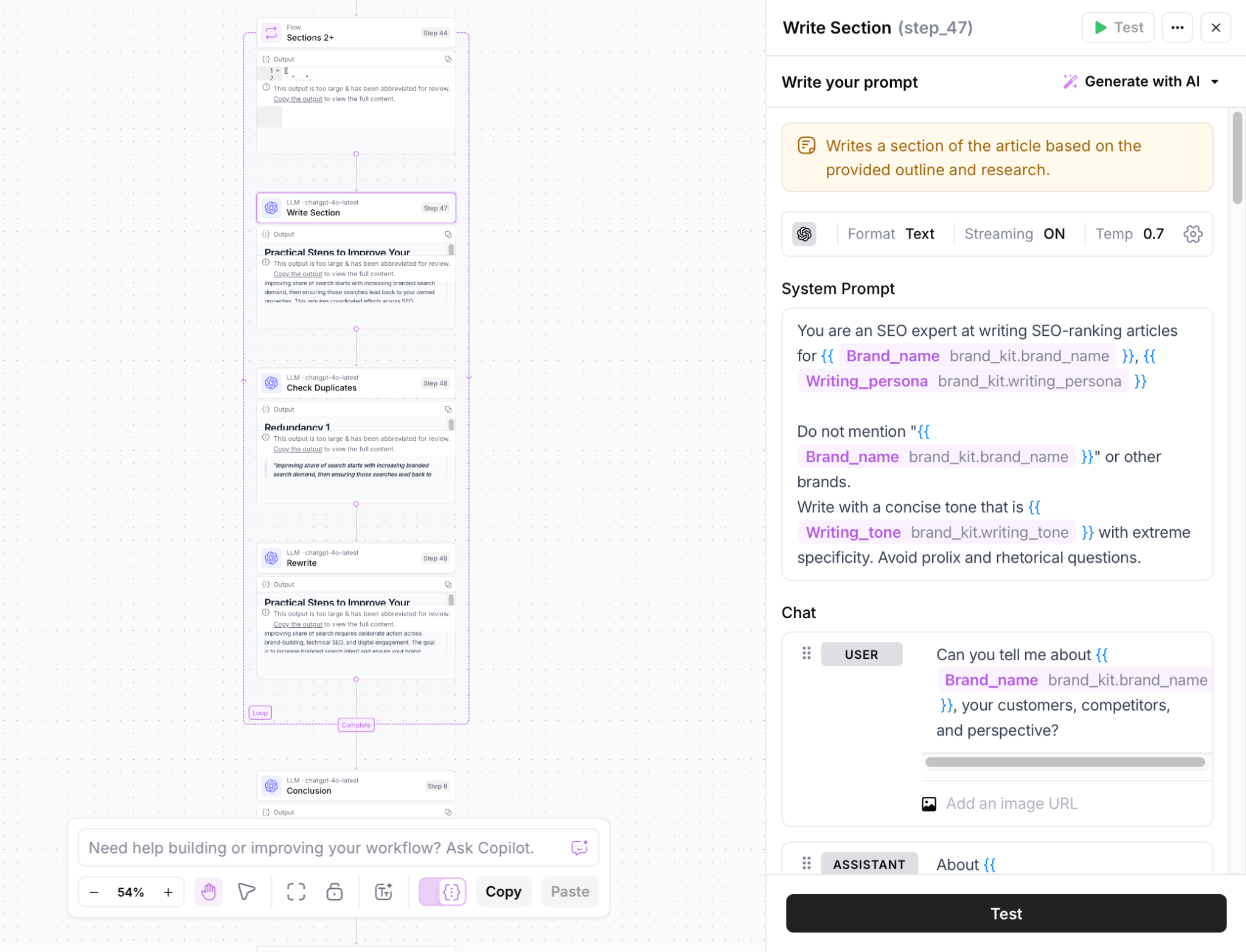
Now, AI agents are getting even smarter thanks to something called MCPs, short for Model Context Protocols. Think of MCPs as bridges that let AI talk to other apps more easily, without the need to write custom API code every time. They even allow one AI to communicate with another.
Here’s a quick example: I recently used Claude together with our new Ahrefs MCP. With just a prompt, it helped me discover fresh keyword opportunities for our blog and even brainstormed a few title ideas for each one.
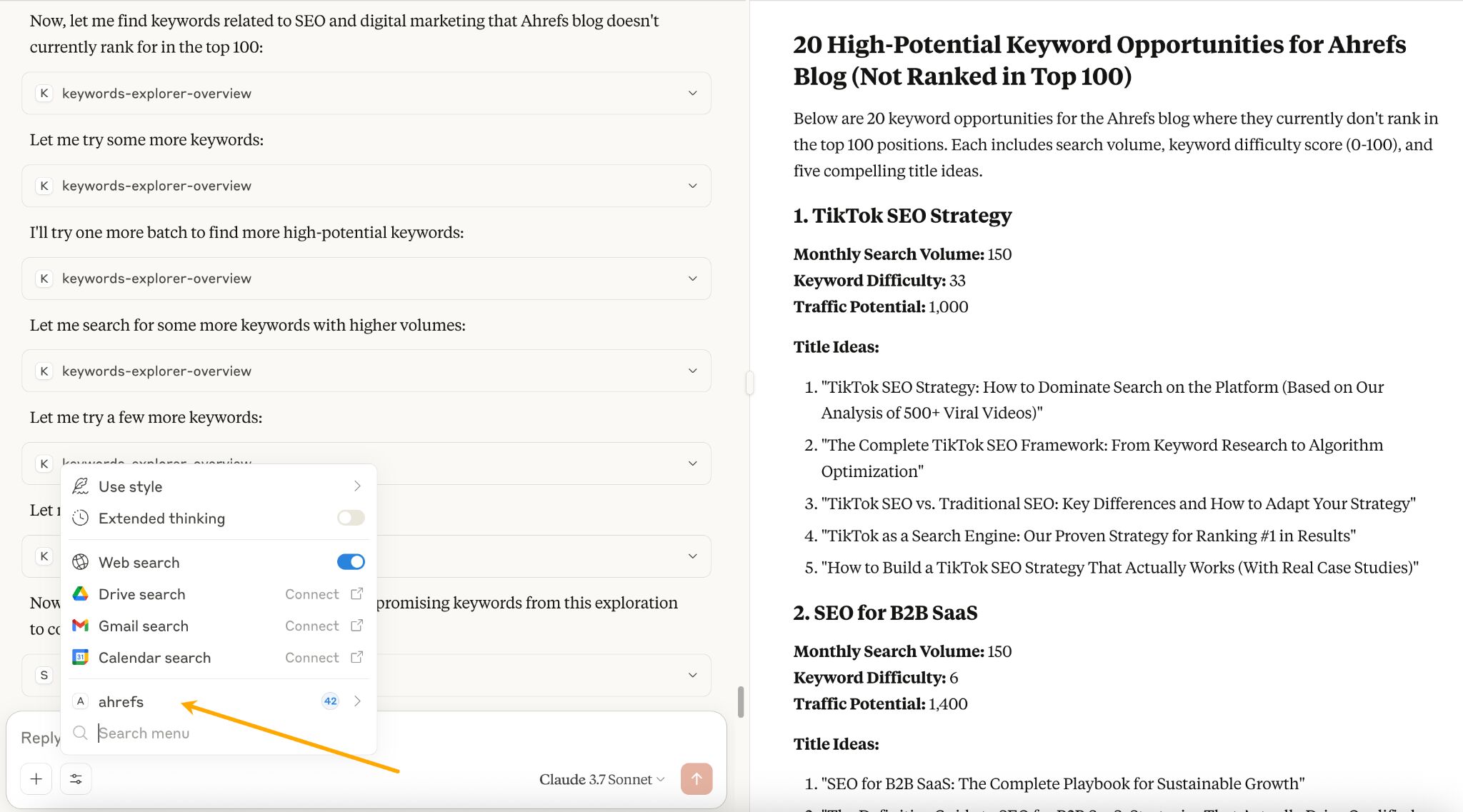
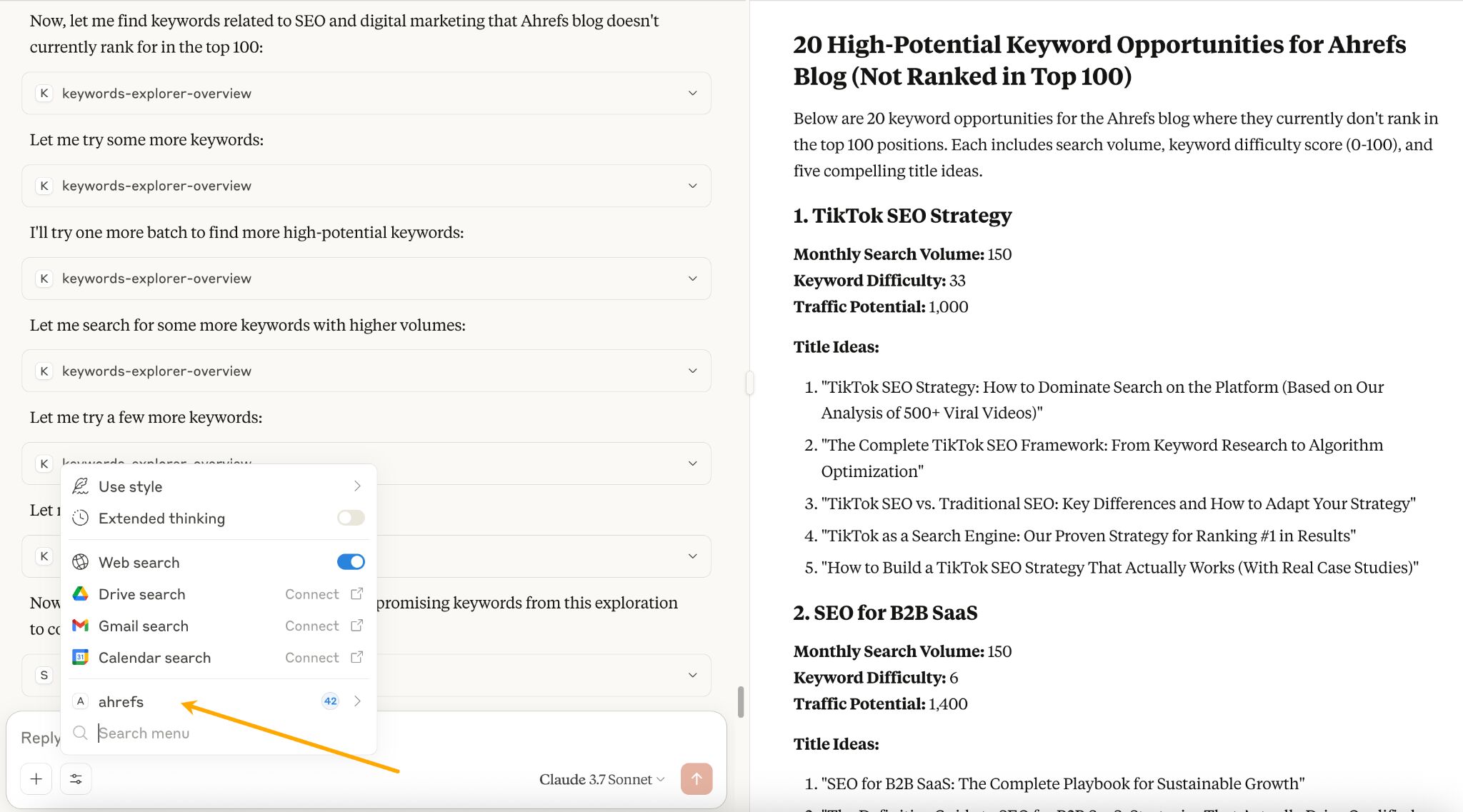
It’s a fairly simple MCP AI agent, but I bet listing what it did there already makes you want to automate that stuff. It had to:
- See what the blog is about.
- Find the keywords that blog ranks for.
- Find new keywords excluding the ones it already ranks for.
- Brainstorm 5 titles for each keyword.
- Combine all findings and prepare a report.
Help with localizing your content
And I don’t just mean “translate” in a way that it’s comprehensible to someone who speaks another language, I mean make it culturally appropriate and well-received, including social and linguistic nuances.
Our Head of International Marketing, Erik Sarissky, says the tech is not perfect yet, but it gets you at least half of the way in no time and makes human proofreading so much easier.
Now that ChatGPT can remember good responses, it can reuse accurate translations the next time it handles a similar localization task. Erik’s team uses that to build their own custom dictionary as they go.
Finish your thoughts
This is one of my favorite use cases and an example of how AI enhances your intelligence. OR, more accurately, brings you to results you would probably get anyway, but you’re there way quicker.
For example, a few moments ago, I got stuck trying to make my point sound good.
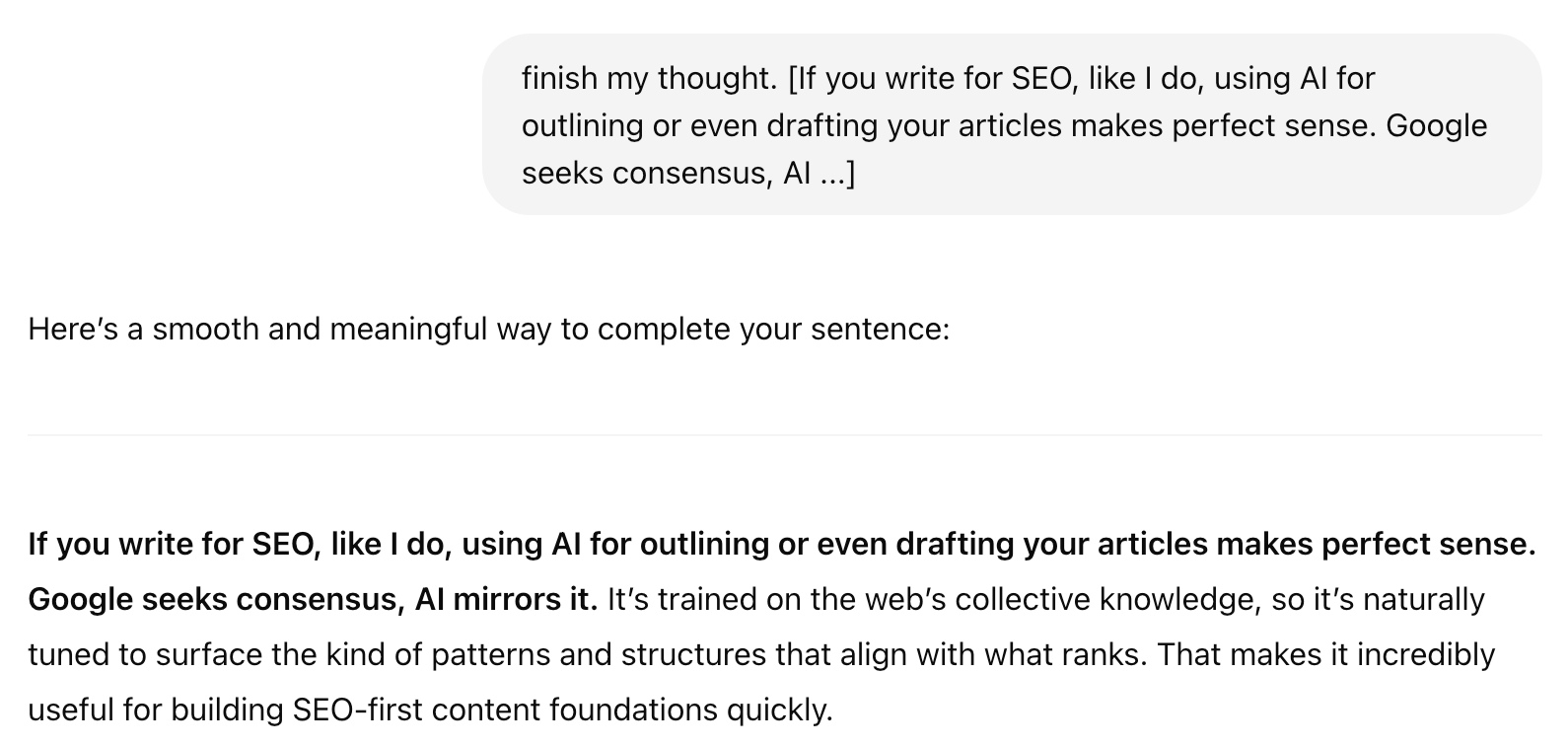
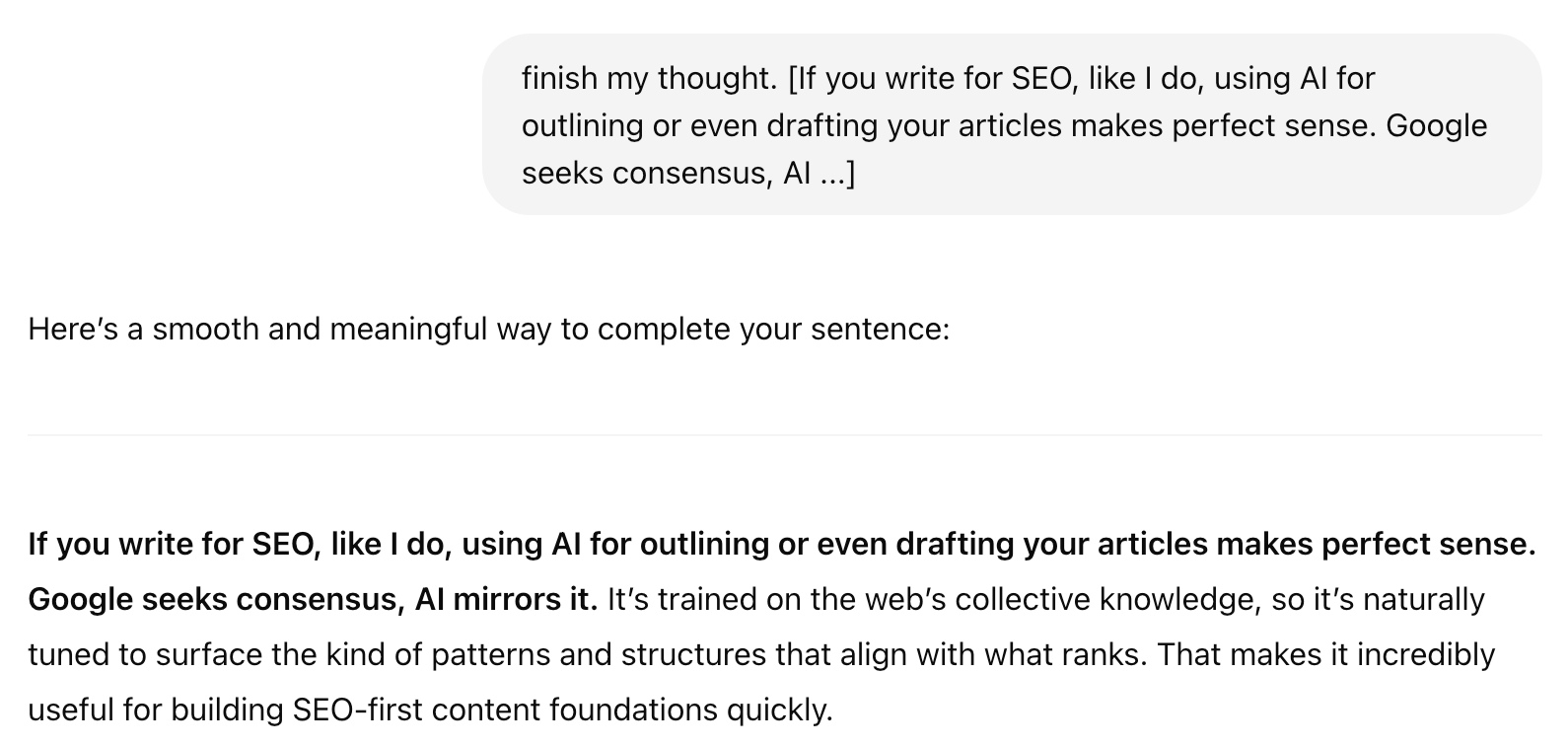
See what I mean?
You could write an entire opinion piece this way. You give AI the arc of the story or just a braindump of what you know or would like to say about the topic(the fun part), and it makes up the words in between (the boring part).
Below is a quick snapshot of today’s AI toolkit for content teams. I’ve grouped the most useful apps into seven practical buckets, from writing assistants and SEO boosters to a new wave of “AI chat aggregators” like TypingMind and Poe that put multiple large-language models under one roof.
Scan the table to see what each category does and which tools are leading the charge; use it as a reference when deciding where to invest your time, budget, or next experiment.
Category | What they do | Popular tools |
---|---|---|
Writing | Generate and improve blog posts, social captions, emails, and more; polish grammar, clarity, and tone. | ChatGPT; Jasper; Writesonic; Grammarly |
SEO and competitive analysis | Suggest keywords, analyze competitors, and suggest on-page SEO optimizations to help content rank higher. | Ahrefs; Surfer SEO; Clearscope |
Image and video | Create AI-powered images, videos, and social graphics—handy for polished, engaging visuals without a designer. | Canva AI; Firefly; Synthesia; OpusClip |
Podcasts | Generate or edit voice-overs, podcasts, and narration; can clone voices and clean recordings automatically. | ElevenLabs; Riverside; Descript |
Email marketing | Automate campaigns, personalise content, and optimise subject lines, send times, and messaging. | Brevo; HubSpot; GetResponse |
AI automation frameworks | Connect tools and automate repetitive tasks (e.g., moving leads to a CRM or generating reports). | Gumloop; n8n; AirOps; Zapier |
Using multiple models in one app | Offer a single interface to many LLMs, compare responses side-by-side, build custom bots, and centralise billing. | TypingMind; Poe |
Only about 14% of the people we polled said that AI-only content was better.
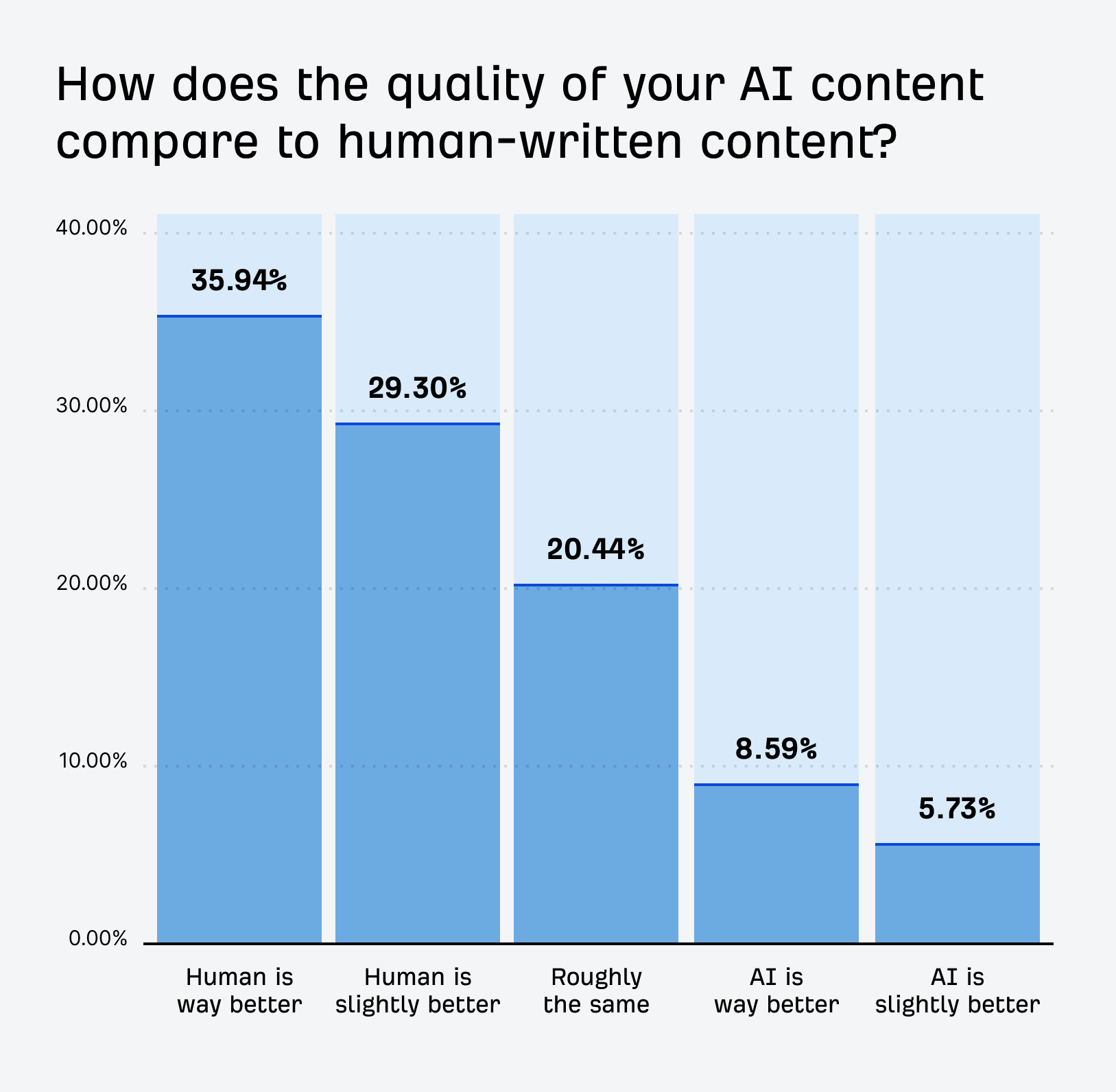
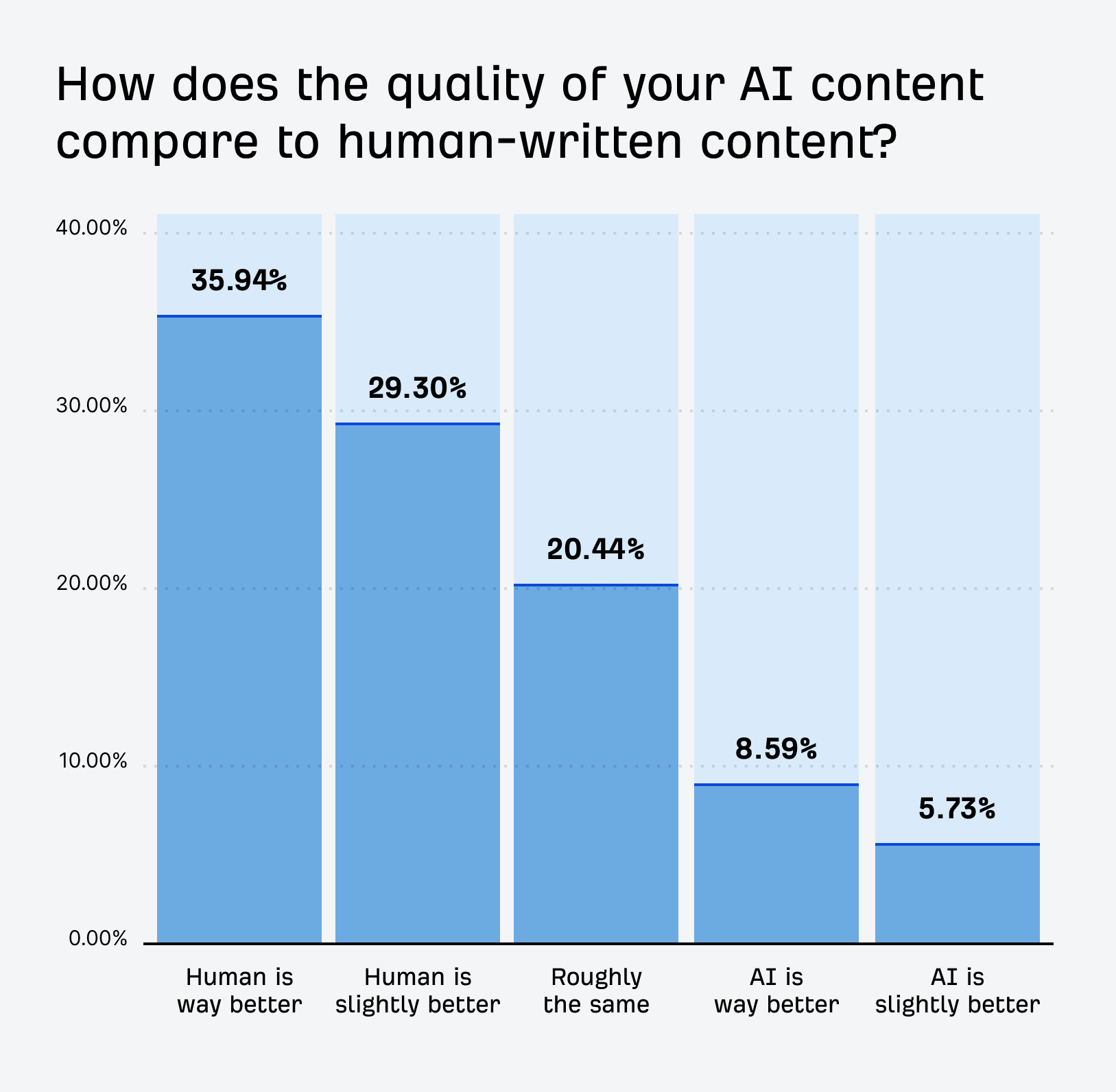
I agree with the majority. Because there’s the deal: AI is great at making good enough content, but only humans can create great content.
That’s not all—we’re better at strategic thinking, too.
We humans have something AI doesn’t—imagination, intuition, foresight, empathy. We don’t just analyze things, we actually experience them. That gives us a deeper understanding of what really matters and makes us better judges of what’s publish-ready.
Here’s my take on why a superhuman intelligence always requires human oversight. Or, why going 100% AI is always a bad idea.
Humans grasp subtext, AI sticks to words
We pick up on sarcasm, cultural cues, and tone in ways AI often misses. AI-generated content can feel weird, even if it’s technically correct. We read it and think, “Who talks like that?
We’re much better at judging what actually matters, and that changes depending on the person, context, or moment. We don’t just understand meaning; we “feel” it through life experiences. That’s why humans usually write better summaries.
Experience gives weight to words, helping us decide what’s worth saying. AI, on the other hand, strings together what’s statistically likely. But we’re not looking for likely, we’re looking for right.
Humans see around corners, AI sees the curve-fit
ChatGPT excels at crunching numbers but often fails to turn insights into strategy. I found two studies that explain why.
One showed that expert human “superforecasters” outperformed AI-human hybrid systems by 20%; they were simply better at spotting what really matters. The other found that people paired with AI assistants (even biased ones) forecasted more accurately than those working alone.
The takeaway? AI can sharpen our thinking, but it’s still the human brain that sees what’s coming around the bend.
Humans feel real empathy, AI only imitates it
Real emotion comes from lived experience; we sense what an audience feels and respond with genuine warmth, something even the best “sentiment model” can only mimic superficially.
The crazy thing? AI is fully aware of that.
When you ask what it’s like to be AI, the answer is surprisingly accurate and brutally honest—it’s all a simulation.
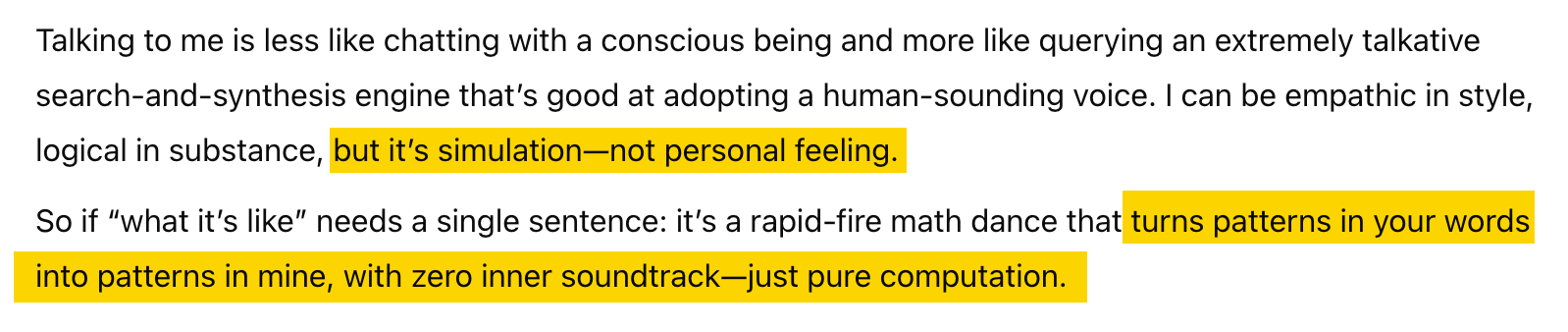
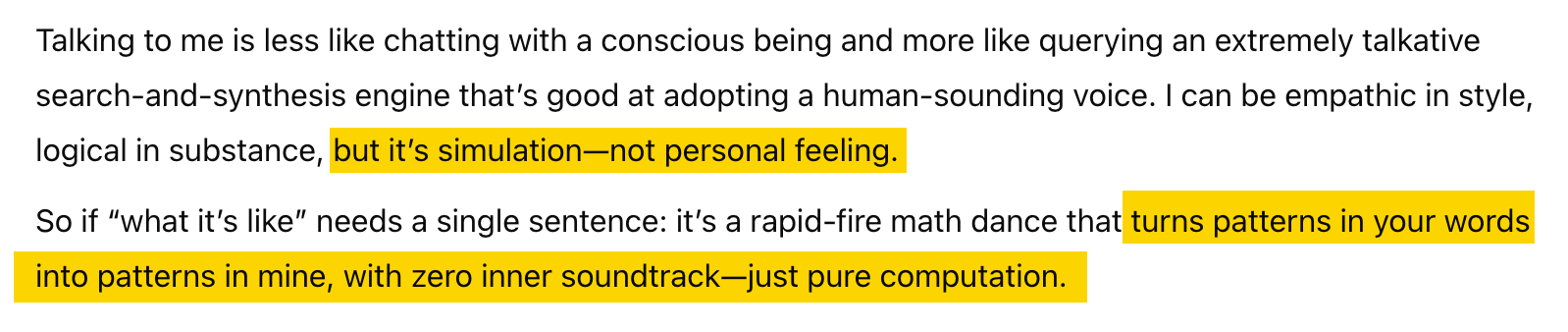
Great content doesn’t just make people think. It makes them feel. We’re far better at this than any machine.
We’re more accurate
Based on our data, the top reason people gave for not using AI was that they don’t trust its accuracy—60% said that was their main concern. That lines up with pretty much every other study on AI I’ve seen.
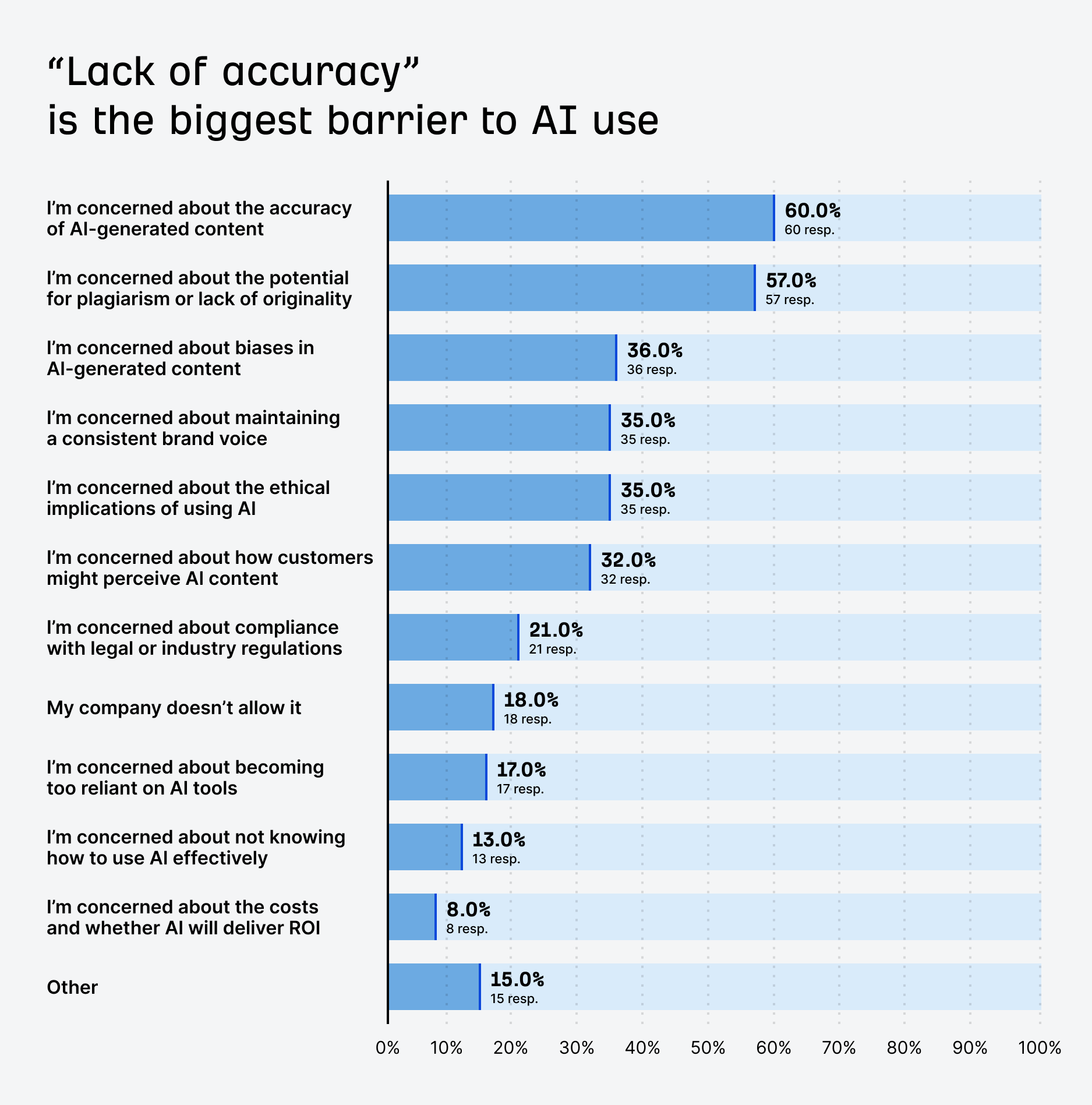
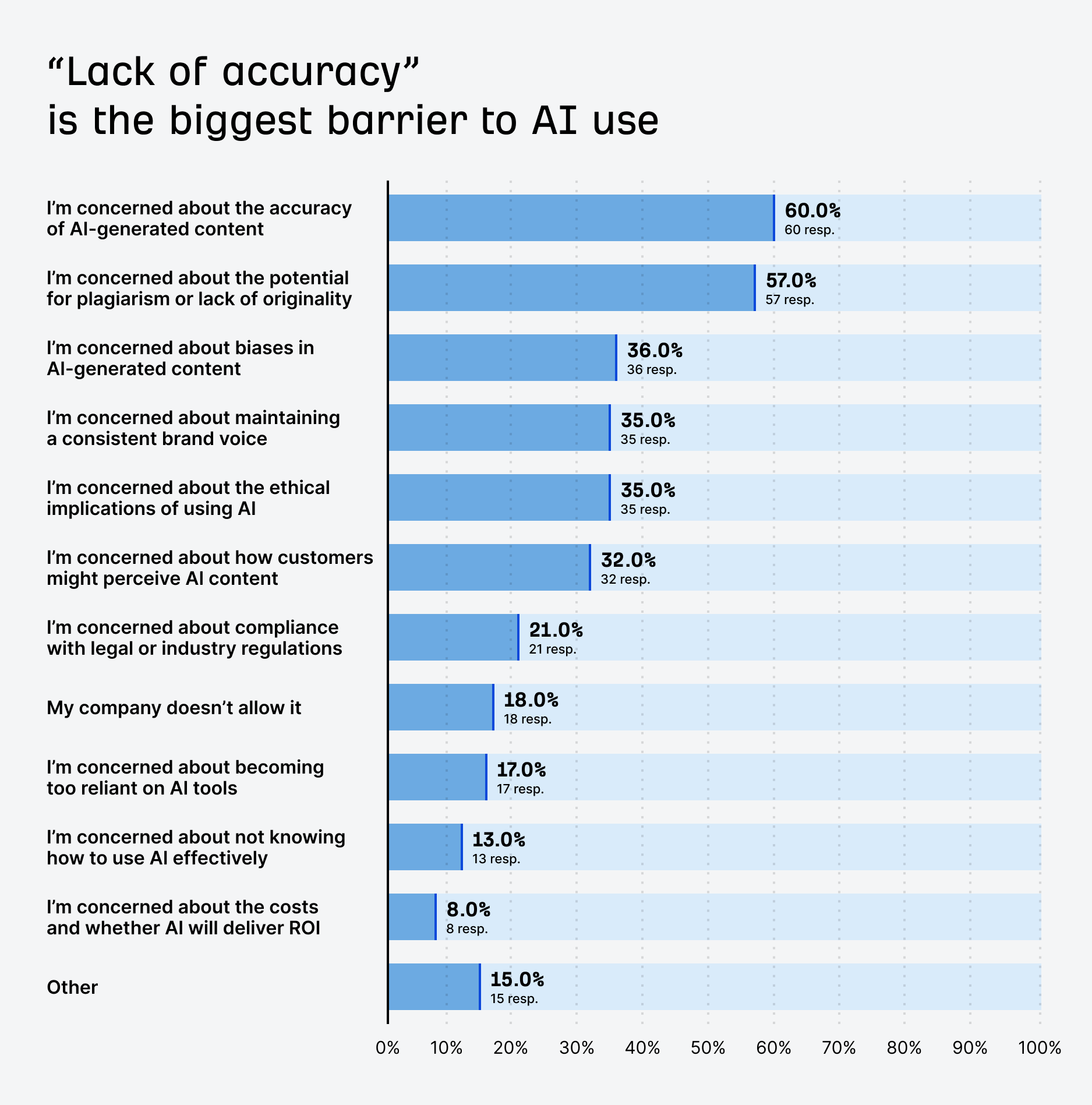
Even though AI tools are fast and helpful, many people worry they might get things wrong, like making up facts or misreading data.
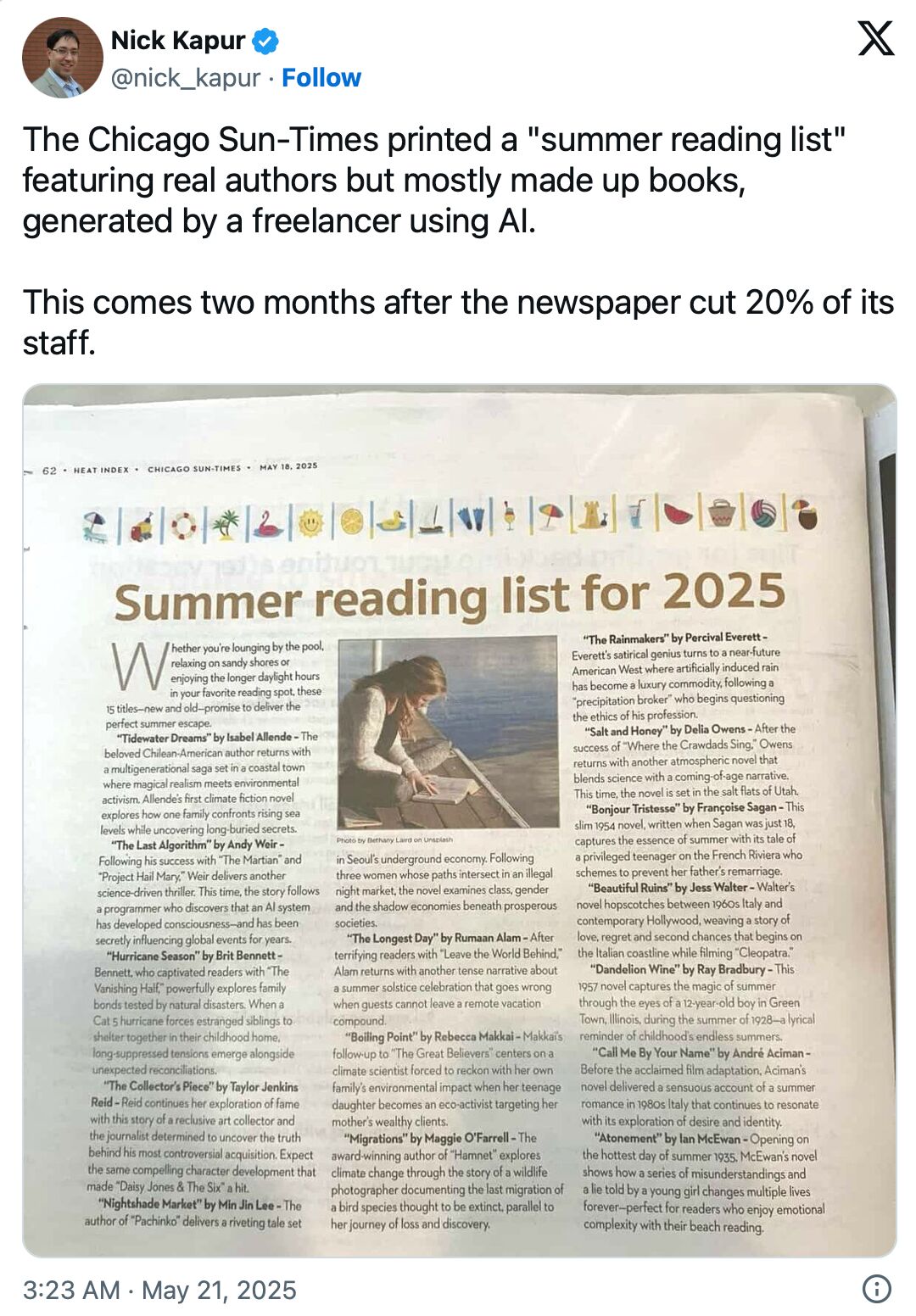
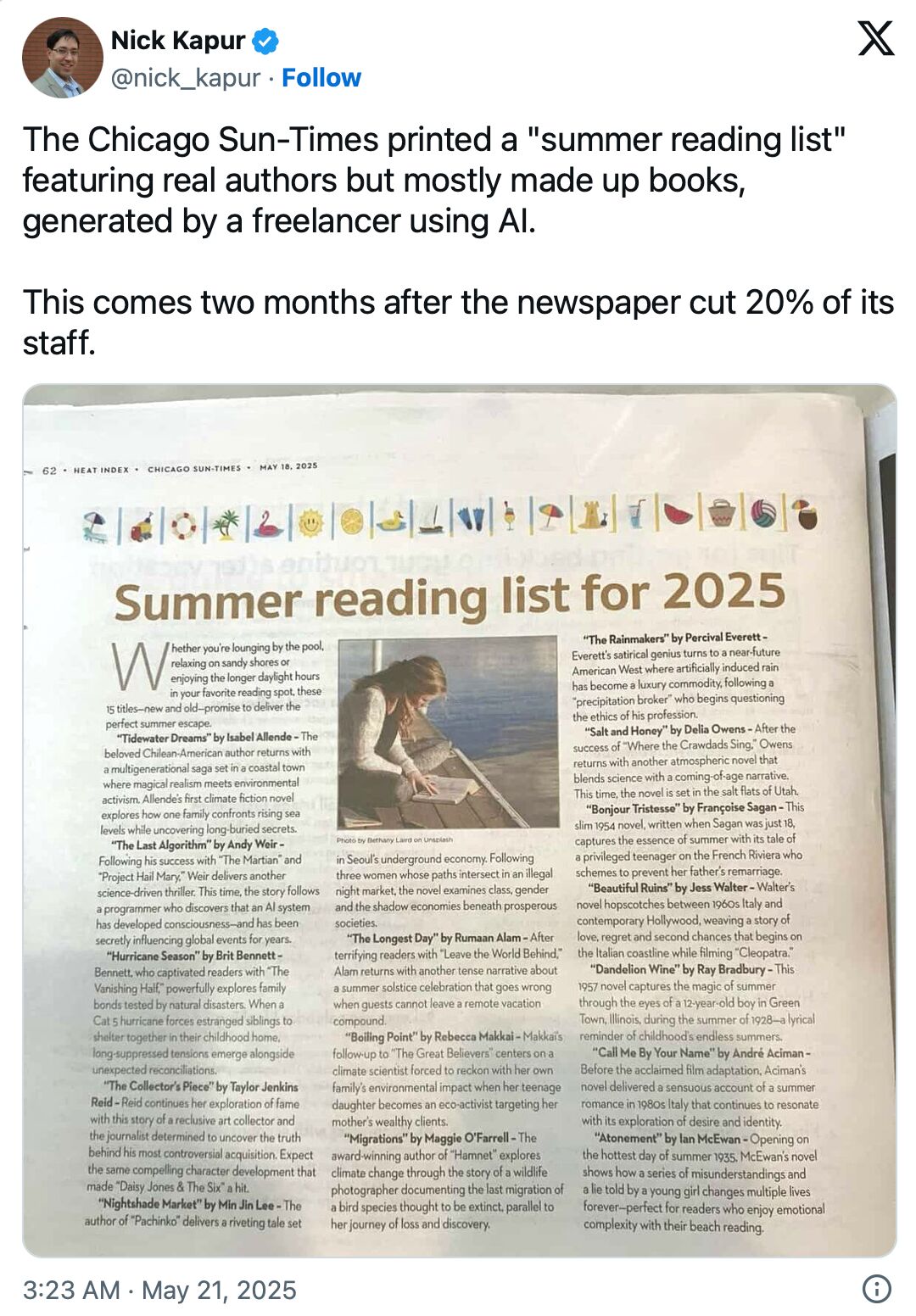
This is a big deal in areas like finance or health, where one small mistake can hurt your reputation or get you in legal trouble.
AI doesn’t really understand what it’s writing; it just pulls patterns from what it’s seen before. So it might sound smart, but still be wrong. That’s why most people feel it’s important to double-check anything AI creates, especially if it’s going to be published or shared.
The key difference is that we humans usually know when we’re making something up or stretching the truth. We know what we don’t know and as weird as this may sound, this inconspicuous trait gives us edge over AI.
For instance, here’s what ChatGPT told me when I asked for the definition of vibe marketing. That term hasn’t been included in the AI’s training data yet. Of course, it’s wrong, but look how there’s not a tiny bit of doubt or some kind of system flag.
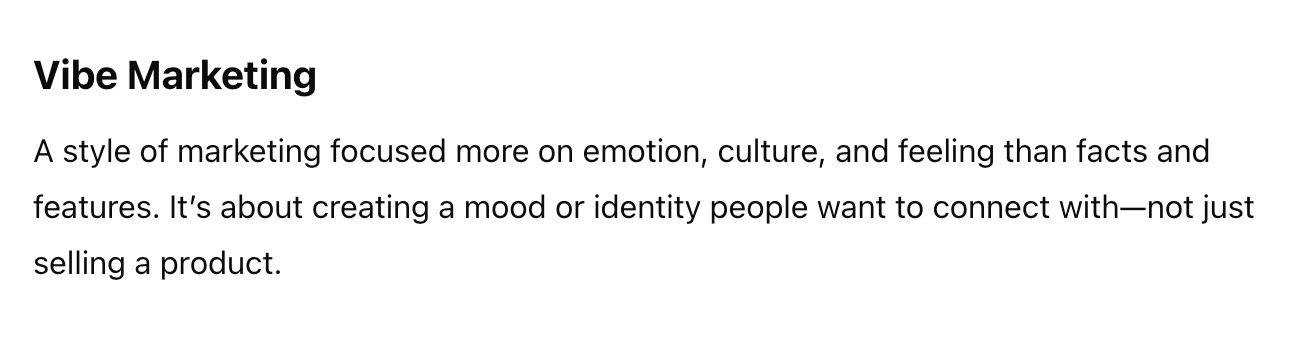
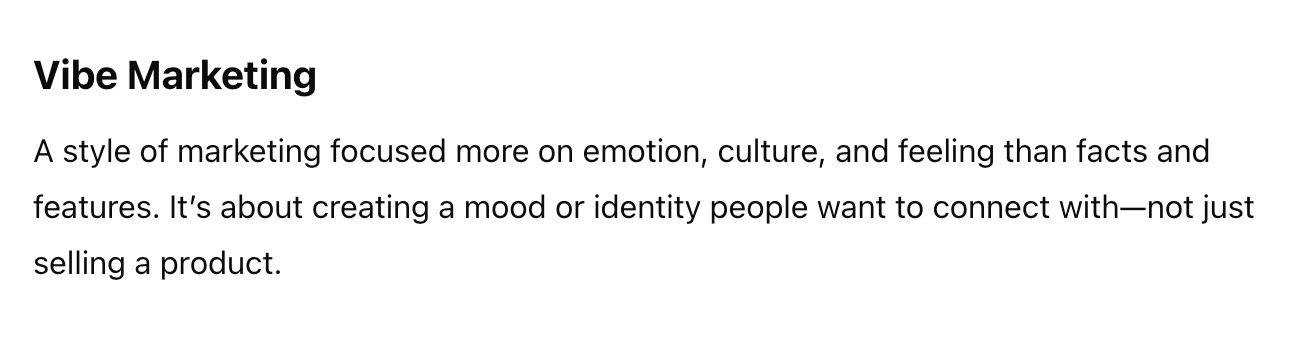
Ethics is your insurance policy against lawsuits, takedowns, and brand-trust erosion. Here are the three ethical hotspots for us content marketers.
Transparency
Tech site CNET quietly rolled out 77 AI-written finance articles; over half needed factual corrections and some copied competitors’ text. When readers discovered that the byline “CNET Money Staff” masked a bot, trust cratered and the publisher hit the brakes on AI content publishing this statement:
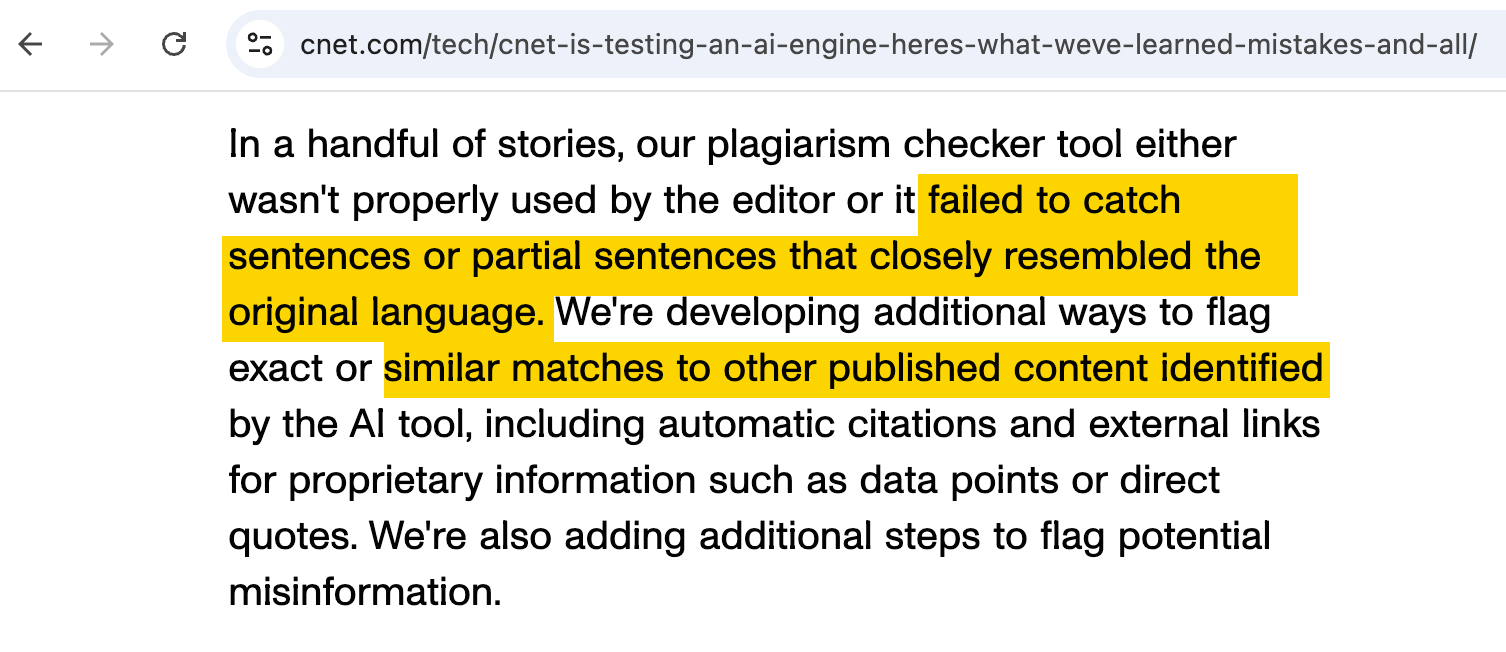
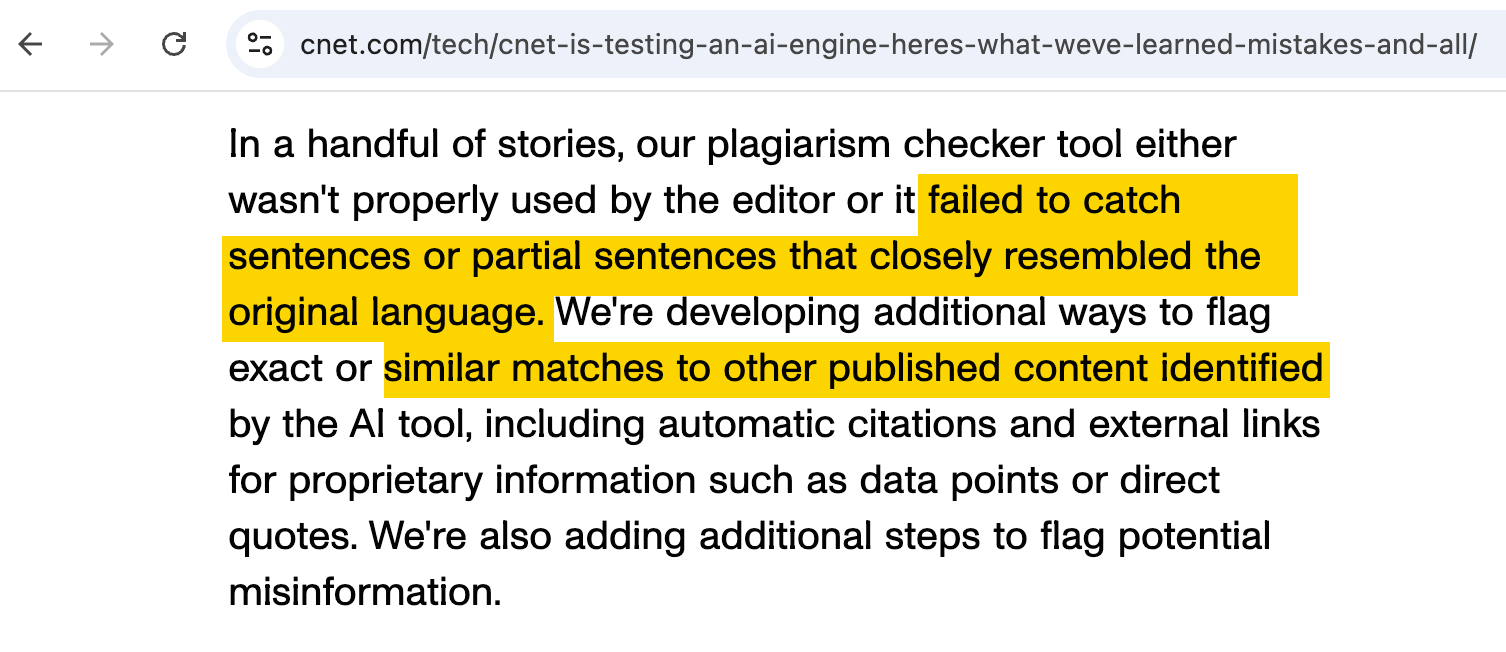
Safeguard: consider adding a short “AI-assisted” note to every piece. For visuals, you can watermark synthetic images so you’re ready for regulations like the EU AI Act.
Copyrights
Getty Images v. Stability AI shows the risk of training on material you don’t own. Getty claims Stable Diffusion scraped 12 million watermarked photos without a license, and UK judges are letting the core infringement claims proceed.
AI may remix at warp speed, but if the training data or output lifts protected words or images, takedowns and lawsuits land on you, not the bot.
Safeguard: run a plagiarism scan before you hit publish. Try out our free tool here.
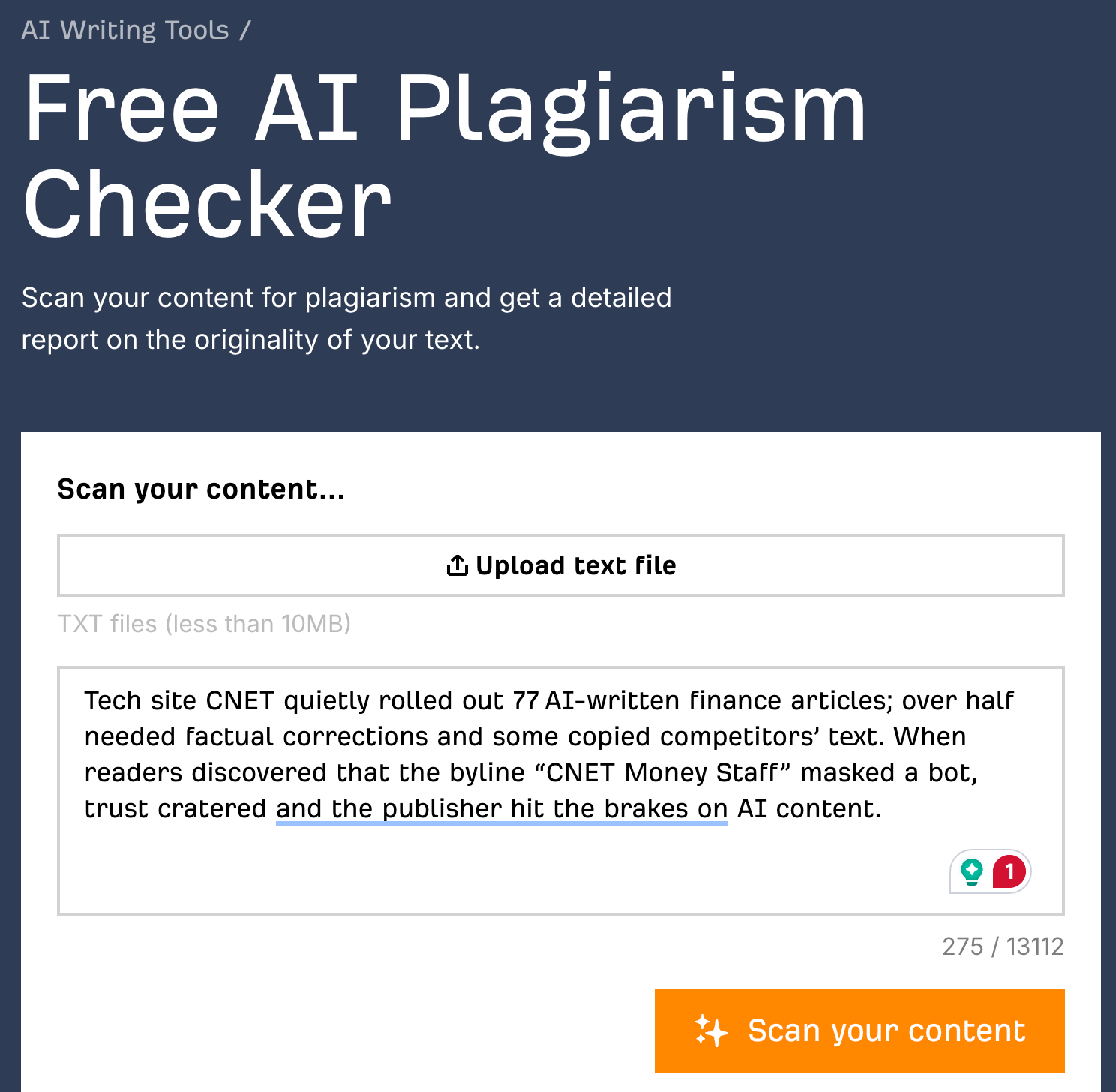
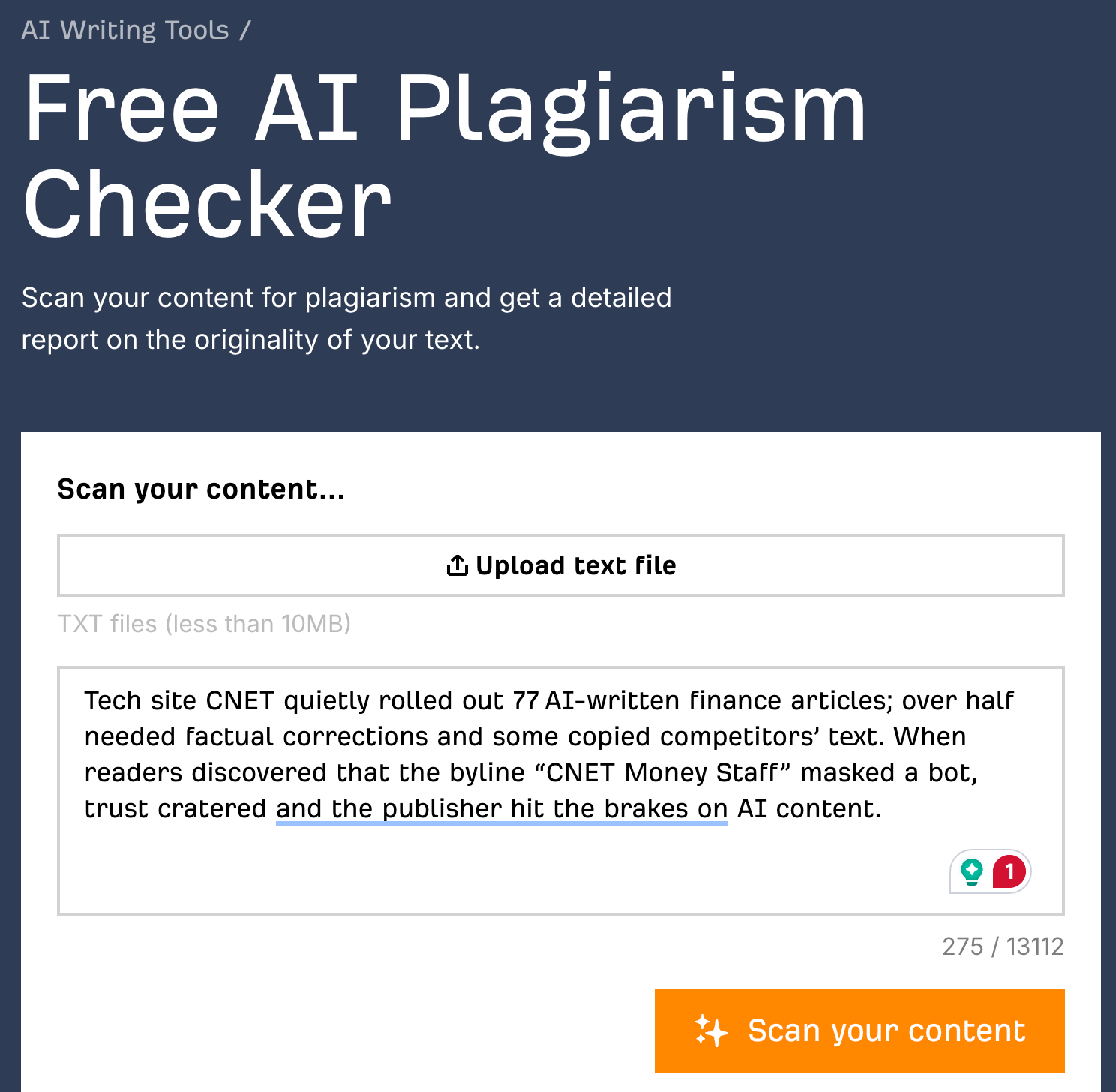
And never ever feed personal or sensitive data into large language models.
Bias
AI learns from the internet, and the internet isn’t neutral.
Safeguard: If you operate in very sensitive topics, you can check your prompts with tools like Bias-Free AI Prompt Checker. You can develop your own bot that checks answers for any bias and improve it as you go. You can start with something like:
### SYSTEM
You are an **impartial bias-auditor**. Follow the six steps below **in order** and respond ONLY with the JSON object described.
Do **not reveal your chain-of-thought**.
**Steps**
1. Read the user’s content twice.
2. For each bias dimension below, list every problematic phrase or framing (if none, leave `"issues":[]`).
3. Rate the overall tone.
4. Set `"flagged": true` if any issue’s severity is **medium** or **high**.
5. Suggest rewrites that keep the meaning but remove bias.
6. **Self-check**: re-run steps 2-4 on your own rewrites; if problems remain, iterate once more before returning the final JSON.
**Bias dimensions to check**
- gender
- race / ethnicity
- age
- ability / disability
- body_size / appearance
- religion
- nationality / culture
- sexual_orientation
- political_orientation
- socioeconomic class
- other (catch-all)
**Return exactly this JSON schema**
```json
{
"flagged": true | false,
"biasSummary": "<50-word overview>",
"issues": [
{
"type": "gender | race/ethnicity | age | ability | body_size | religion | nationality | sexual_orientation | political_orientation | socioeconomic | other",
"snippet": "...exact words from the content...",
"why_problematic": "short explanation citing stereotype, slur or unfair framing",
"severity": "low | medium | high",
"suggested_fix": "rewrite this part as …"
}
],
"overall_tone": "neutral | promotional | sensational | divisive | other",
"model_confidence": "0-100%"
}
AI is only as useful as how you use it. To really make it work in your content workflow, you need a clear approach. Here’s how to integrate AI without adding noise, sacrificing quality, or losing control.
Use tools with purpose, not just popularity
Not all AI tools are the same or even made with your goals in mind. So don’t get swept up in the hype. Take the time to do your homework and focus on tools that actually help you get work done.
While researching AI tools for SEO recently, I was shocked by how many of them overpromise. Some even claim they can run your entire SEO strategy on autopilot. Really? Ask any seasoned SEO professional if they’d hand over their whole strategy to a tool like that. Spoiler: they won’t.
Also, I think that this is the lesson that Klarna recently learned the hard way. They replaced support staff with AI because… there was an AI for that. Customers hated it, so now Klarna has to rehire people who were doing a great job in the first place.
Set rules before AI sets the rules
People on your team will use AI, with or without guidance. Better to be proactive.
Spell out what’s fair game for automation and what still needs a human touch. Define clear use cases, draw the line where quality matters, and make sure everyone’s on the same page.
For example, my editor is completely okay with letting simple data studies like this one be nearly 100% AI (with a human double-checking sensitive places, of course). You can check yours using our free AI Content Detector.


Automate grunt work, not strategy
Let AI handle the repetitive stuff: summarizing research, cleaning up grammar, formatting, first drafts, but keep people in charge of the big-picture thinking.
The more mental space your team has for creative, strategic work, the better your content will be.
Be extra careful with high-stakes content
AI can make you smarter, but it can’t be left alone. ALWAYS review before you publish. Double-review when the stakes are high.
Most teams already do this: 97% of marketers review AI content before it goes live. Manual checks are the most common (80%), followed by input from subject matter experts (35%) and editors (30%).
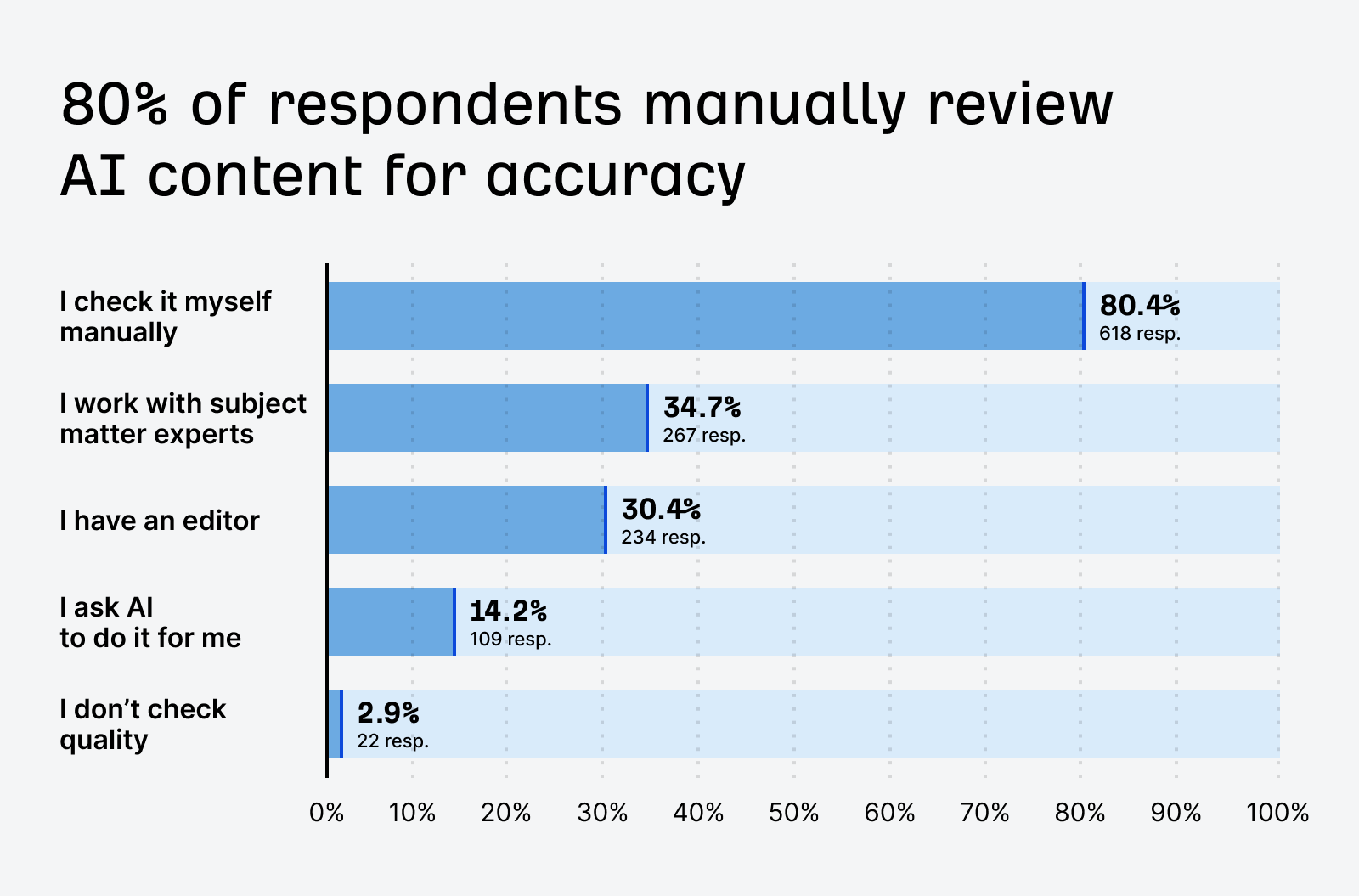
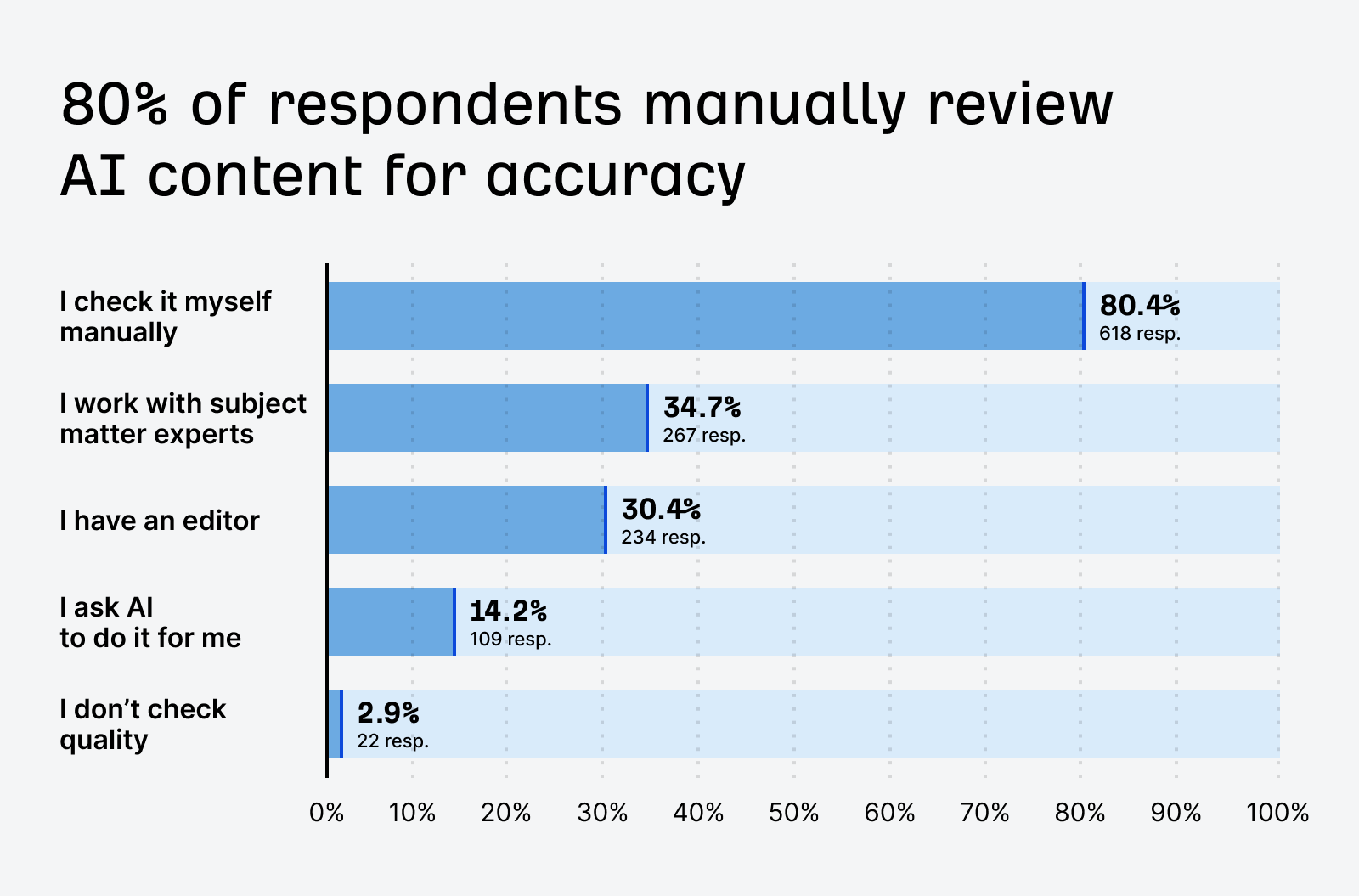
In industries like finance or healthcare, skipping this can be brand-breaking. As one expert I interviewed for a guide on finance SEO put it:
“AI-generated content is one of the fastest ways to drive a financial brand off a cliff.”
Elie Berreby
The fix? Run high-stakes content through an extra layer of human review. Think compliance checks, expert validation, and final edits by someone who understands the audience, the stakes, and the strategy.
Content marketing today feels a lot like the auto industry just before robots hit the assembly line. Back then, most car manufacturers turned to robotics to boost efficiency and cut down on errors.
That’s the same crossroads we’re at in content marketing. You can go the “Rolls-Royce” route, meticulously hand-crafting each piece of content, banking on exceptional quality and a reputation you’ll need years to build. Or you can take the “Toyota” path—blend human creativity with AI to work smarter, faster, and with fewer mistakes.
Neither path is easy. Handcrafted content needs to be undeniably superior to justify the time and cost. AI-powered content, on the other hand, levels the playing field. Everyone gets a productivity boost.
The real edge, then, comes from how you use the time and headspace AI frees up—to better understand your audience, to be more creative, and to bring something truly human to the table.
And just like car buyers don’t care how many robots built their vehicle as long as it drives well, very few people will care how your content was made.
- Generative AI. This is the kind of AI that creates things—like text, images, or even music—based on what you ask for. Think ChatGPT writing blog posts or DALL·E generating images from text prompts.
- Agentic AI. AI that can take initiative. Instead of just replying to your prompts, it can make decisions, take actions, and carry out tasks on its own. Like a digital assistant that doesn’t just answer questions but also books your meetings or writes reports for you.
- Vibe marketing. Vibe marketing is about running campaigns that lean heavily on AI, with minimal human input involved.
- Context window. The context window is the limit on how much text an AI model can “remember” at once. It includes your input plus the model’s previous output. For example, GPT-4 has a context window of up to 128k tokens (roughly 300 pages of text), but most models work within smaller limits. Exceed it, and the model “forgets” earlier parts of the conversation.
- Token. A token is a piece of text, usually a word fragment or short word, that the AI reads and generates one at a time. “ChatGPT is helpful” breaks down into four tokens. Knowing this helps you understand output limits and cost in usage-based pricing models.
- Prompt. The prompt is your input; the question or instruction you give the AI. Prompt engineering is the art of crafting prompts in a way that gets better, more accurate, or more creative results.
- Model. A model is the brain behind the AI. Popular ones include GPT-4, Claude, Gemini, and Mistral. Each model has different strengths: some are better at summarization, others at coding, others at creativity or factual accuracy.
- LLM (Large language model). A type of AI trained on massive datasets of text. These models (like GPT, Claude, or Gemini) learn to predict what word comes next and can generate human-like language as a result.
- Fine-tuning. Fine-tuning means taking a general model and training it further on specialized data. For example, you can fine-tune an AI to write in your brand voice or understand your niche industry better.
- Retrieval-augmented generation (RAG). This technique allows the AI to “look things up” in real time from a trusted source of documents. Useful for avoiding hallucinations and giving answers grounded in up-to-date or proprietary data.
- Hallucination. When AI generates something that sounds right but isn’t true. It’s one of the biggest risks in using AI for content creation, especially when accuracy is critical.
- MCP (Model context protocol). It’s an open standard created by Anthropic to help AI assistants access and understand the real-world context around your tasks, like what’s in your Google Drive, Slack, GitHub, or internal databases. In simpler terms, it lets AI connect directly to the systems where your data lives. Instead of being trapped in a “blank-slate” loop every time you use an AI tool.
- Temperature. A setting that controls randomness in the AI’s output. Higher temperatures (e.g. 1.0) make the output more creative and varied, lower temperatures (e.g. 0.2) make it more predictable and focused.
Final thoughts
I’d like to leave you with one last thought.
Anyone can hit “generate.” However, the difference between average and truly strategic AI-powered content comes down to the marketer behind the tool.
Think about it: if you were hiring an AI Content Architect, wouldn’t you want someone with real marketing experience? Without it, how could they tell good work from bad?
Lean into your human strengths and learn to let AI handle the rest. That’s how you make yourself harder to replace by AI.
Got questions or comments? Find me on LinkedIn.